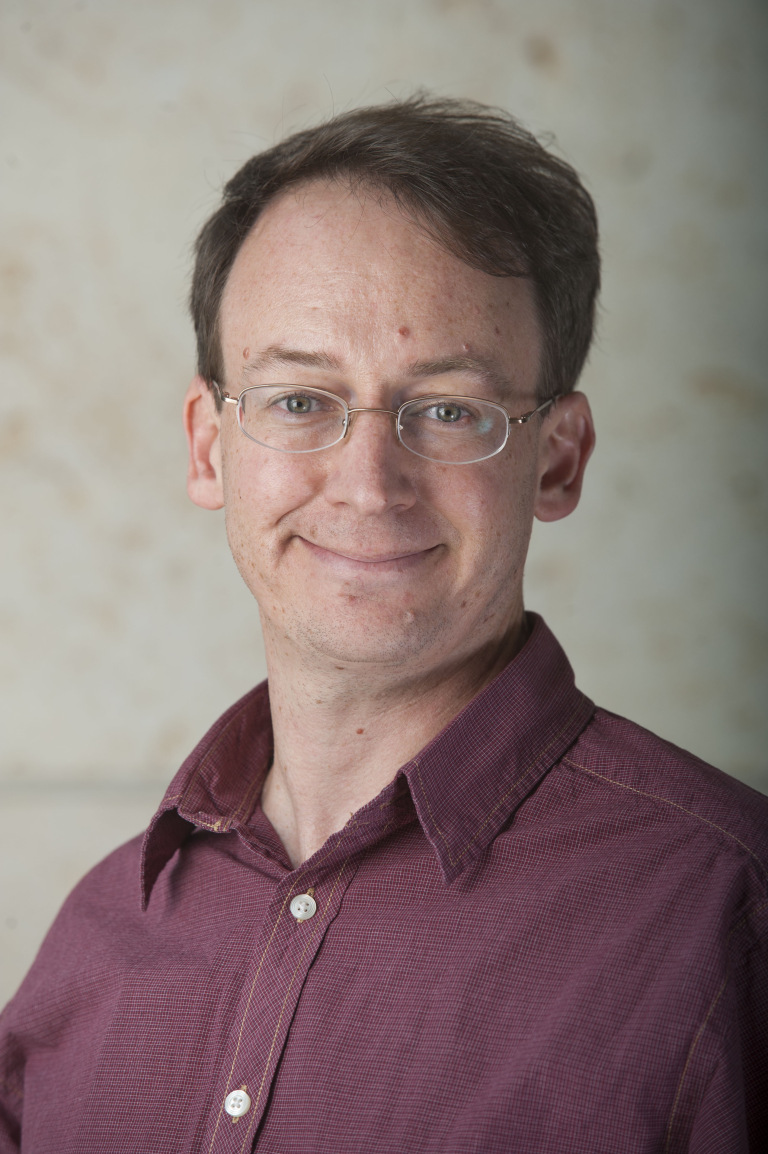
When an ecologist realized he’d made a fatal error in a 2009 paper, he did the right thing: He immediately contacted the journal (Evolutionary Ecology Research) to ask for a retraction. But he didn’t stop there: He wrote a detailed blog post outlining how he learned — in October 2016, after a colleague couldn’t recreate his data — he had misused a statistical tool (using R programing), which ended up negating his findings entirely. We spoke to Daniel Bolnick at the University of Texas at Austin (and an early career scientist at the Howard Hughes Medical Institute) about what went wrong with his paper “Diet similarity declines with morphological distance between conspecific individuals,” and why he chose to be so forthright about it.
Retraction Watch: You raise a good point in your explanation of what went wrong with the statistical analysis: Eyeballing the data, they didn’t look significant. But when you plugged in the numbers (it turns out, incorrectly), they were significant – albeit weakly. So you reported the result. Did this teach you the importance of trusting your gut, and the so-called “eye-test” when looking at data?
Daniel Bolnick: Only partly. The fact is, there really can be cases where trends may be weak but significant. Or, real trends may exist after controlling for many other variables, and therefore a plot of y ~ f(x) might look like a shotgun scatter, when in fact y does depend on x after variable(s) z are accounted for. In other words, 2-dimensional plots might fail to capture actual trends. So I still do believe that our eye-test, while useful, is not a sufficient basis for judgement. That’s why we do statistics. We just need to do the statistics correctly.
RW: So your supposed result – that animals that are phenotypically more similar have more similar diets – turns out to be not true. Does that surprise you, given that the assumption was that they were?
DB: Absolutely, it surprises me. I do think that I was predisposed to accept the “significant” trend despite my “eye-test” being negative, precisely because I really truly expected this result to hold. In fact, I still believe it holds, and I just need to do a better job of measuring diet or morphology. I have other data, published elsewhere, that still leads me to strongly suspect the phenomenon holds true, even if it didn’t show up with this particular method in this particular population/year.
RW: Is this your first retraction? If so, how did it feel?
DB: Yes. It felt horrid. A sinking feeling in my gut, and I had a hard time sleeping that night. Once I found the mistake, I wrote to the journal immediately. Their official retraction notice is coming out in the next issue.
RW: We noticed the journal removed the paper entirely – which goes against the retraction guidelines issued by the Committee on Publication Ethics. Is that why you decided to write the blog?
DB: No – and I have a copy of the paper, for anyone who wants to read it.
RW: You write about your mistake and the ultimate retraction – something you didn’t enjoy doing, as you note: “It certainly hurt my pride to send that retraction in, as it stings to write this essay, which I consider a form of penance.” Most researchers don’t write public blog entries when they retract papers – why did you choose to do so?
DB: It was my penance. 500 years ago I might have walked through the town square whipping my back. This seems a bit more civilized, and preferable. Actually, what interested me most in writing the blog post was the notion of errors in statistical code. The R programming language has taken the biology world by storm. It’s what cool kids use. I’ve always considered myself a bit of an “R-vangelist”. In that regard, I’ve argued a lot (in a very friendly way) with Andrew Hendry who runs the blog in question, who hasn’t taken to R. This experience gives me pause, a bit. But really it is a mixed lesson. I only figured this out because I saved my R code and could retrace every step of every analytical decision, to find a mistake. That’s a good thing about R. But it happened because I, like many others, wasn’t an expert programmer in general, or in R in particular at the time. So the reliance on R predisposes us to these kinds of mistakes. The lesson here is that R is a two-edged sword, we have to be careful with it. That’s what I wanted people to learn, to avoid future mistakes.
RW: You note that other researchers make similar statistical errors, which should ideally be checked during review. Yet you admit that requires time and expertise on the part of reviewers, which we don’t always have. So what’s the solution, in your opinion?
DB: I’m taking over as Editor-In-Chief of The American Naturalist in January 2018, the oldest scientific journal in the US. So your question touches on something I’m thinking a lot about from a practical standpoint. Here are the barriers, as I see it:
- Not every researcher uses statistical tools that leave a complete record of every step, in order. Given the potential problems with coding errors, we shouldn’t require people to do so. That means this probably can’t be an obligatory part of review.
- Any journal that stuck its neck out and required well-annotated reproducible code + data for the review process would just see its submissions plummet. This needs to be coordinated among many top journals.
- Reviewers would either say “no” to review requests more often, or do a cursory job more often, if we required that they review R code. And many great reviewers don’t know how to review code properly either.
Solutions, perhaps:
- Make this optional at first. To create an incentive, we put some sort of seal-of-approval on papers that went through code-review. As a reader, I’ll trust a paper more, and be more likely to cite it, if it has that seal. Authors will want it. Readers will value it.
- Find a special category of reviewer / associate editor who can check code. May be separate from the regular review process and may not require the subject matter expertise. The easiest way to do this is to hire someone, and charge authors a small fee to have their paper checked to get the seal-of-approval.
- A halfway version is to require code be provided with the data during review and upon publication, both. No formal review of the code is required, but reviewers MIGHT opt to do so. That creates just enough fear in the authors to create an incentive to proof-read and well-annotate their own code. They may find errors in doing so. Basically the proof-reading goes back to the authors, but we entice them to self-proof-read a bit more carefully than they otherwise might do.
Like Retraction Watch? Consider making a tax-deductible contribution to support our growth. You can also follow us on Twitter, like us on Facebook, add us to your RSS reader, sign up on our homepage for an email every time there’s a new post, or subscribe to our daily digest. Click here to review our Comments Policy. For a sneak peek at what we’re working on, click here.
I like idea #3- b/c you can implement it fairly easily. Ideally someone would check code but b/c of practicality and cost, #3 seems a reasonable approach. I am sure this step will be automated soon enough anyways.
And bravo to the author- I can imagine it is a horrible feeling to know you have published incorrect data BUT considering your finding was wrong and would have insignificant- this “finding” and result is sort of bonus. We need an annual award for integrity in research, and I know of suitable nominee.
If he thinks that reviewers go through every step of the code in reviewing an article, he would be way wrong. This is the responsibility of the author. Authors should check their code, and if they are not good at coding, ask for help from a colleague. It is not possible for reviewers to get at that level of detail. Having worked in SAS with the code of others, it is never easy, and can be very time consuming. Reviewers do not get paid to do this job.
I don’t think this is what he is suggesting. Rather, he suggests specialist reviewers solely for the code. A bit like some of the specialist reviewers often used for statistics by a number of journals. They only look at the statistics and whether it was done right.
OK, who’s gonna volunteer for that? Journals have a lot of problems getting reviewers. This requires a specialized reviewer who will spend more time on the code. I am not volunteering for that. Would you?
Don’t know, maybe the same type of people who are currently reviewing statistics for journals?
So agree with you. Why should a reviewer be expected to go through each and everything of the paper to approve its validity. As a reviewer my job is to see if the results are novel enough to justify publication and if the authors have done a decent job to having adequate data to support their claim. As a reviewer, how on earth am I expected to comb through everything. Its not just about R code, in general, every technique even in experimental labs if not done correctly will give you skewed data and its up to the author to judge that. Even if I have an expert in a particualar method/technique, its nearly impossible for the reviewer to judge if “everything” was done correctly.
I think we are stretching is too much
I agree with including the code for examination IF THE REVIEWER WISHES TO DO SO. However, it is a more extreme situation to get a specific reviewer to go through the code. If we cannot assume that things were done correctly, the entire scientific enterprise falls apart.
First, a bravo to Dr. Bolnick for what was obviously a painful admission, both personally and professionally. While I’m sure this has a negative tint for him, I do believe his actions on this have only increased his professional prestige in the long run, in addition to providing an excellent precedent for others, especially young researchers. So again, thank you.
I also respond to add to the informative discussion here and on the blog post. On the subject of R, you are correct in being skeptical of authors abilities to use the software/procedures appropriately. There is an additional danger not mentioned, however. The R ecosystem is replete with user written packages. Even when the packages are correctly applied, users should assess their accuracy and correctness. One very large advantage of commercial statistical software such as SAS, is the thorough vetting it goes through before release. The procedures there are typically designed by statisticians/mathematicians (often those who have developed the relevant statistical theory themselves) and the software is written by teams of professional coders who intimately understand the coding process. In R, it is not unusual for a user to have little idea of a package author’s abilities. Even when that ability exists, there is no guarantee that the package has been maintained and documented properly. I don’t mean to discourage the use of R here. In fact, I am currently using it myself for a large project. I would just caution users to investigate the background of R packages they choose, especially those involving newer cutting edge procedures. Have the authors published on the package? Is it widely used? Has it been maintained? Can the examples be replicated in other software? Answers to these questions can give users more confidence in the results they obtain.
Lastly, I would recommend a change to your corrected title. Rather than saying “individuals are NOT correlated”, I would suggest a more tentative version that “No correlation was found between individuals …” As you describe above, there may be confounding factors masking a potential relationship. All you can really say is that with this data, and this analysis, you haven’t been able to identify a relationship. It’s the old mantra, “Never accept the null hypothesis” 🙂
Again, thank you for speaking and writing on this retraction. It is a positive move.
Bill Price
While I applaud the offer for quickly retracting the article in question, does this not further the problem of publication bias? Would the correct action not be to issue a correction, correct the statistics and report in non-significant finding? Perhaps this may be less of an issue in this field, but non-positive results are equally as important to the positive ones, and should be reported…
Yes and no. We can only hope a journal will publish a “non-significant” finding, but a correction usually signals that the conclusion still holds. That’s not the case here, so a retraction would appear appropriate.
I worked as a computer programmer for a local government agency for a few years before going to grad school. Our activities dealt with money, and we were thoroughly trained to be extremely careful with everything we did, including extensive checks to make sure things were working correctly, thorough program testing, and extensive documentation. I was kind of astonished in grad school to see just how cavalier people were with their data and programs, given that this was effectively their ‘currency.’ I continue to see numerous examples of mistakes and problems having to do with misunderstandings, low skill levels and lack of attention to testing and checking, I have to concur with the Bill Price’s comments above about the need for caution, and I would say extreme caution, with R procedures that are user-written and not necessarily correct or correct for your application. You are much better off sticking with something like SAS.
I find your trust in quality control of commercial software packages a bit strange. Yes, R packages are written by users (by the way, who are the SAS programmers and why is the quality of their code guaranteed to be better?), but at least everyone can check open source code. Btw., Dr. Bolnick’s mistake could just as easily have happened with any other software as it apparently was a failure of understanding documentation: “I clearly thought that the Mantel test function in R, […], reported the cumulative probability rather than the extreme tail probability.”
Trust in commercial packages is not anymore strange than trust in any other aspect of modern life. There are good reasons for trusting certain entities. For example, in flying across the country, I put far more trust in an aircraft conceived, designed, built, and tested by a team of professional engineers and mechanics over one built by the guy down the street in his garage who happens to like model airplanes. No, there are not any guarantees, but the associated risks involving mishap are greatly diminished.
SAS programmers are professional coders, explicitly trained and educated in that field, typically being diverse in multiple operating systems and languages. They work in teams, using strict programming protocols, under the direction of senior programmers, statisticians and mathematicians who understand the theoretical aspects of the analyses and procedures in question. Code is vetted multiple times, at multiple stages, internally before enduring several layers of beta testing externally. Procedures are extensively documented in minute detail and thoroughly referenced allowing those with the know-how to examine the behavior for anomalies. When such anomalies occur (and, yes, they have), SAS has a large, and highly competent, technical support system available who will contact the developers, if required, to address any problems. In software development circles, SAS is often regarded as a gold standard to be modeled. All this happens because, as a business, quality performance in the field is essential for the continued success and reputation of that business.
Of course any software can be misused or misunderstood. Show me any scientific journal, from any time, and I can be certain to find multiple examples of such. I don’t believe CarolunS, and certainly not myself, meant to imply otherwise. The message is simply cautionary, not exclusionary.
Those are the same arguments I’ve seen in debates of Windows vs Linux or Wikipedia vs traditional encyclopedias. They are based on a false dichotomy, i.e. that Open Source precludes doing the very same quality control measures. In fact, if the user base is big enough (as it is for the more successful R packages) Open Source has the advantage because more experts scrutinize the code. And even less widely used packages are usually written.by the experts who developed the method and, again, you can check the code for errors.
SAS has a huge number of folks whose job it is to test code, to ensure that code is backward compatible, to resolve issues. R has none of those. You basically trust folks to do the right thing, and you have no idea whatsoever if they have done so. Doug Bates is on record as saying that it is not his job to resolve discrepancies between his program and the comparable program in SAS. As an R user, it falls on you to ensure that the code works as it should. In SAS, the corporation takes that responsibility.
Code is part of the Materials and Methods. Omitting the code or omitting the commands used in the command line is omitting part of the methods. Without that, that study cannot be reproduced, and has no business being published. You cannot simply reduce the methods to say “Trust us, we knew what we were doing.”
That being said, I view two problems. As someone above had posed, whose responsibility is that? This cannot be the responsibility of the author, since the whole point of peer review is to have an outsider examine the paper to consider both validity and novelty. This means someone with expertise in this field, both understanding the theory but also the methods, so they could verify if they would expect some method to give the results that were presented.
This brings the second problem, which is a more general issue of peer review. Finding reviewers is time consuming, and so is reviewing a paper. For this reason, it is difficult to get more than two people. Then to have to find even more people who are actually experts in all of the discussed topics, which for a very large paper, may mean multiple very different experiments (imagine population genetics in humans to identify some disease locus, and then making a mouse model). Having two people review a paper is not a good strategy in general, and perhaps this means our entire peer review system is not structured correctly.
So in a more practical sense, I would agree to a compromise. Not all analysis is done by people with a lot of experience in writing code, and it shouldn’t have to be. Often we have to try many analyses to get something that works, or is intuitive to use, or gives output in a way we can understand. For the second idea, of specialist reviewers, I wouldn’t want to see sloppy but correct code being the source of a rejection. So, it probably should be required to provide the code, much like the raw data as well, and the code should be in a format (such as a script) so that one could run the code on the data and generate some figure exactly as it was in the paper. Regenerating the data from source would potentially be an easy way for reviewers to verify the code.
It’s been a few years, and Dr. Bolnick has certainly had some, shall we say, more experience with journal retractions. I would be interested to hear a follow-up from him regarding some of his thoughts about extra processes to bring to a journal.
Did he ever try any of these? If not, why not?
Did they work?
Has his opinion on these suggestions changed?
How will AI affect this discussion, in, let’s say, 1-year. Honest question!