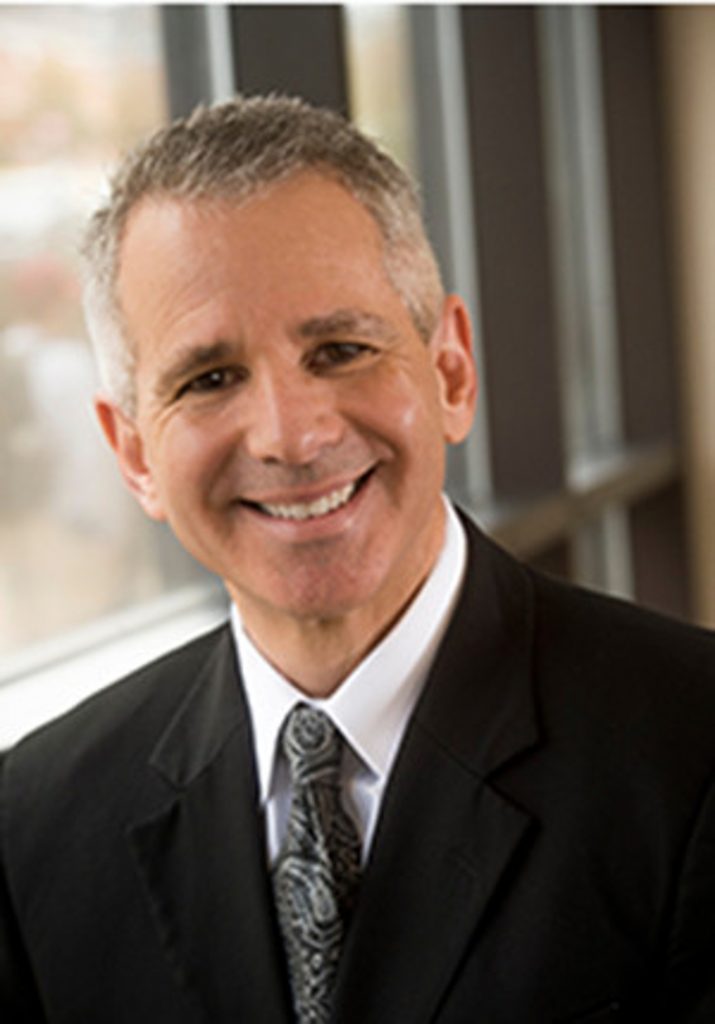
The work of David Allison and his colleagues may be familiar to Retraction Watch readers. Allison was the researcher — then at the University of Alabama, Birmingham, now at Indiana University — who led an effort to correct the nutrition literature a few years ago. He and his colleagues are back, this time with what might be called the “Regression to the Mean Project,” an attempt to fix a problem that seems to vex many clinical trials. You may have noticed some items in Weekend Reads about letters to the editor that mention the issue. Here, Allison explains.
Retraction Watch (RW): First, what is “regression to the mean,” and what does it mean for clinical studies?
David Allison (DA): Regression to the mean (RTM) is a ubiquitous statistical phenomenon, just as, for example, sampling variance is a ubiquitous phenomenon. “RTM is a statistical phenomenon that occurs with any pair of variables that have a correlation not equal to |1.0|. With RTM, the subjects’ average values for an outcome variable (e.g., BMI) change in a systematic direction over time despite there being no treatment effect.” The mere existence of RTM is not a criticism of any study, similar to the existence of sampling variance. However, drawing inferences (conclusions) without accounting for the effects of these phenomena is problematic. It is exactly these cases that we have drawn attention to and have written about.
In clinical studies, RTM is observed when baseline and follow-up measurements are not perfectly correlated (i.e., when the correlation coefficient ρ≠1). Specifically, when measures of baseline values such as BMI are measured again at the end of the study, the average follow-up value in the subgroup that had the lowest values at baseline will rise, while the average follow-up measurement value in the subgroup that had the highest values at baseline will fall, even in untreated subjects. These changes from baseline to follow-up are often misinterpreted as an intervention effect. (To be more precise, a number of qualifying statements about overall population distributions would have to be offered. These can be found in standard texts or formal statistical papers on the topic, but we capture the gist of the issue here.)
Bias due to RTM can be accounted for by including a control group in the study design.
RW: What’s an example of how failing to take regression to the mean into account in a study can have an effect on the results?
DA: Although there are multiple ways in which failing to take RTM into account can lead to mistaken inferences, three seem most common.
In the simplest case, investigators evaluate individuals from the population who hold extreme measures in a study without a control group. For example, in childhood obesity intervention studies, where we see this commonly, investigators study children with overweight or obesity at baseline, who are usually defined as having baseline BMI values some level above the population mean. Then, as would be expected solely by RTM, at follow-up weeks, months, or years later, the children on average have BMI values that deviate less from the population mean of children at the subsequent time point. Note that this does not necessarily mean that the children’s absolute BMIs went down (although they may). Rather, it means that their BMIs, on average, are fewer standard deviations from the mean at the second time point than they were at the first. The common use of ‘BMI z-scores’ in childhood obesity studies may make this inferential error easier to miss. Most investigators understand that without a control group there are potential explanations for changes in the intervention group other than the intervention itself, but may not appreciate that the observed results are actually expected, due to RTM. For an example from the world of sports, consider “The Sports Illustrated Cover Jinx.”
In a more complex case, subjects are selected as described above, but a control or comparison group is included. The authors do not observe a statistically significant difference in the change in outcome from baseline between the two (or more) groups, but do observe that all groups pooled in one sample had a statistically significant change in the outcome from baseline. They miss the point that the change (incorrectly labeled as the ‘effect’) that occurs in a pooled sample is also what would be expected under RTM from a single sample.
Finally, the case that seems most pernicious is this: The investigator finds no statistically significant evidence of change in a group or of treatment effect overall, but then divides the overall sample into subsets on the basis of the baseline values of the outcome variable. The investigator then analyzes each subset separately and finds that the subsets with the highest baseline values experienced the greatest reductions and that within that group, the reduction was statistically significant. The investigator may even go on to show that the subsets with the lowest baseline values experienced statistically significant increases in the outcome and may argue that the treatment ‘normalizes’ the population, that is, pulling in both tails of the distribution toward more ‘normal,’ healthy values. If this were true, the overall variance of the distribution would have to decrease, but this would still not necessarily be evidence of a treatment effect. The pattern of results is, again, exactly what we would expect under RTM, i.e., even with no treatment at all.
RW: Is this a problem most nutrition researchers are aware of?
DA: RTM and other similar statistical phenomena were most likely included in most researchers’ statistics education. I think investigators remember or, even if they did not study them, intuitively ‘get’ most of the classic threats to validity in pre-post designs without control groups laid out by Campbell and Stanley in 1963, including such things as maturation. But RTM (which Campbell and Stanley referred to as ‘statistical regression’ or just ‘regression’) does not seem to be fully appreciated by many investigators. Even those who do know of it often think it only relates to situations involving substantial measurement error. They may think that for variables like BMI which can be measured precisely, RTM is not an issue. Yet as Campbell and Stanley stated, “While regression has been discussed here in terms of errors of measurement, it is more generally a function of the degree of correlation; the lower the correlation, the greater the regression toward the mean. The lack of perfect correlation may be due to ‘error’ and/or to systematic sources of variance specific to one or the other measure.” So although RTM may be included in statistics education, exposure to phenomena like RTM tends to not stick as well as do other topics.
RW: How many journals have you written to? What do you think should happen to these papers, and what has the response been?
DA: We have submitted around a dozen papers or letters on RTM to journals. The vast majority have been accepted for publication. However, as we have seen with our prior work on correcting the literature, the process remains challenging, with high variability in journal and author response. One journal did not publish our letter, but then published an excellent correction by the authors that received praise as a model of integrity. At another extreme, a journal declined to publish our letter because the authors personally targeted individuals in their rebuttal. The editor decided to not publish our letters as a pair to avoid publication of the personal attack. While this more extreme outcome was undesirable in our opinion, we still find that most authors respond politely and professionally. Some concede our points, some defend their original conclusions despite our points, and some do not respond. A discussion on one of our letters to the editor highlighted the fact that RTM continues to mislead and cause errors in published research.
RW: Have any of the studies you’ve looked at informed nutrition guidelines or programs?
DA: While we are not aware of whether any of the papers that, in our opinion, made inferential errors by not taking RTM into account were individually dispositive of a policy or program implementation, these papers do contribute to the body of information that can be drawn upon by policy makers, clinicians, and organizational leaders.
One example is the PEACH trial. The PEACH trial was developed with the goal of reducing obesity in young children. Effective strategies to combat the obesity epidemic are much needed worldwide; thus, this study could potentially have a meaningful impact on best practices in policy making for childhood obesity treatment. The original study concluded that their approach achieved the goal of reducing obesity prevalence and that parental involvement should be incorporated as best practices for childhood obesity treatment in community settings across the Australian state of Queensland. Unfortunately, the reductions in child BMI, the study’s primary outcome, are attributed to RTM rather than the PEACH intervention. The more recent extension of the PEACH intervention, which is the subject of our recent letter to the editor, did not include a control group, making the changes in child BMI even less convincing given the lack of comparison group. Thus, in this trial, the results cannot substantiate conclusions of intervention effectiveness or efficacy because of failures to take RTM into account. While we cannot state with confidence that these results have informed policy, they certainly have made an impact in the scientific community. As of October 19, since its publication in 2011, this article has been cited 113 times in Google Scholar, 64 times in Web of Science, and 68 times in Scopus.
In another example, for which a letter has not (yet) been published, the researchers attempted to study the effectiveness of the We Run This City (WRTC) Youth Marathon Program, a youth fitness program implemented by a multi-organization collaboration in Cleveland, Ohio. Even though the authors concluded that the program is effective in ‘improving’ physical fitness among youth and ‘reducing’ BMI in a subgroup of youth with overweight or obesity at baseline, these conclusions are unsubstantiated because the study authors did not account for RTM.
RW: What’s the solution to the “regression to the mean problem?”
DA: As with most things, there is likely no single solution. Statistics education could cover RTM more thoroughly in research methodology courses. The importance of including a control group in research studying intervention effects should be reemphasized. We have constructed a flowchart that can be accessed as a web-based app that we will be submitting for publication soon. The flowchart walks investigators, reviewers, and editors through a decision tree to determine whether RTM is plausibly biasing inference in a study. Researchers can further their education by attending statistical-oriented talks at conferences, taking continuing education courses, viewing videos of short courses offered freely on the University of Alabama at Birmingham’s Nutrition Obesity Research Center website, and other professional development activities.
Allison notes: My principal writing partners on this topic are Drs. Diana M. Thomas of the United States Military Academy and Cynthia Siu of COS & Associates. They have co-authored all our letters to the editor on RTM. Other co-authors on individual letters or papers have included Cynthia Kroeger, Tanya Halliday, Bridget Hannon, Chanaka Kahathuduwa, TaShauna Goldsby, and Asheley Skinner. Each of the aforementioned scholars helped to prepare these Q&A responses.
Like Retraction Watch? You can make a tax-deductible contribution to support our growth, follow us on Twitter, like us on Facebook, add us to your RSS reader, sign up for an email every time there’s a new post (look for the “follow” button at the lower right part of your screen), or subscribe to our daily digest. If you find a retraction that’s not in our database, you can let us know here. For comments or feedback, email us at [email protected].
This pattern of observed responses cries out for a statistical analysis, with due attention to RTM!
See:
Thorndike, R. (1963). Chapter 1: Designing research to study achievement vs. predicted achievement. The concepts of over- and underachievement (pp. 1-24). New York: Bureau of Publications: Teachers College Columbia University.
Students who scored high on standardized IQ tests and lower on the achievement test became “underachievers.” Students who scored high on the achievement test but low on the IQ test became the “overachievers.” Thorndike showed that this phenomenon was inevitable regression to the mean. Both terms took (and maybe still take in some quarters) pejorative connotations. I remember from the early 1960s high-school teachers openly discounting the apparent academic success of female students as overachievement. I think this practice was very common.
I am male and was of low status in K-12, so I was saved by scoring well on the standardized tests. But the tests in the hands of people ignorant of basic statistics did do much damage.
This problem never seems to go away. Here are the references to a letter published by Nieto-Garcia in 1990, 28 years ago, discussing this and referencing the article by Oldham in 1962, 56 years ago. And the same issue is still arising!
1: Nieto-García FJ, Edwards LA. On the spurious correlation between changes in blood pressure and initial values. J Clin Epidemiol. 1990;43(7):727-8.
2: OLDHAM PD. A note on the analysis of repeated measurements of the same subjects. J Chronic Dis. 1962 Oct;15:969-77.
I recommend Kahnemann and Tversky on pilot training, a classic example.