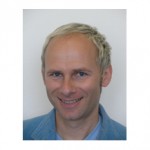
A new group of experts is suggesting there’s something fishy in the body of work of social psychologist Jens Förster.
The University of Amsterdam, Förster’s former employer, commissioned three statistical experts to examine his publication record, looking for signs that the data are not authentic.
Well, they found some signs:
After conducting an extensive statistical analysis, the experts conclude that many of the experiments described in the articles show an exceptionally linear link. This linearity is not only surprising, but often also too good to be true because it is at odds with the random variation within the experiments.
The authors classify the investigated publications into three categories: publications with strong, unclear or no statistical evidence for low veracity.
Of the investigated articles, eight fall within the first category: strong statistical evidence for low veracity. Three articles fall within the second category and four articles in the last.
For more details, you can read the full report here.
Forster has posted a response to the report on his website, some of which we’ve included here:
I will need some time to process the new report that I saw yesterday afternoon for the first time. Because I was sworn to secrecy with respect to the report and the email I received, I also need to figure out how I can defend myself without referring to the contents.
For now, I would like only to express my outrage at the procedure, by which the present report is published without allowing me time to prepare a response. UvA’s intention is completely unclear to me; I do not even know the names of the members of the commission who decided this.
The university plans to send a copy of the report to the 11 journals that fall within the first two categories — showing “strong” or “unclear” evidence of “low veracity” — along with a request for retraction.
Here are some of the papers in the “strong” category of “low veracity” (the full list is in figure 17.1):
- Förster, J. (2011). Journal of Experimental Psychology: General, 140, 364-389.
- Förster, J. (2010). European Journal of Social Psychology, 40, 524-535.
- Förster, J. (2009). Journal of Experimental Psychology, 138, 88-111.
- Förster, J. and Denzler, M. (2012). Social Psychological and Personality Science, 3: 108-117 (Now retracted.)
- Förster, J., Epstude, K., and Ozelsel, A. (2009). Personality and Social Psychology Bulletin, 35: 1479-1491
- Förster, J., Liberman, N., and Shapira, O. (2009). Journal of Experimental Psychology: General, 138: 383-399
Förster has been the subject of two inquiries, and has denied charges of data manipulation. He is now at Ruhr-Universität Bochum in Germany. (He also appears to be planning to teach at a workshop about research ethics.)
We’ve contacted the University of Amsterdam for more information, and will let you know if they respond.
Like Retraction Watch? Consider supporting our growth. You can also follow us on Twitter, like us on Facebook, add us to your RSS reader, and sign up on our homepage for an email every time there’s a new post.
It looks like that Frankfurt workshop is a general research and writing thing, not specifically about ethics. So rather than inviting Förster to speak at an ethics workshop, they brought him in to a general workshop so that he can give a talk about ethics, since I guess that’s a specialty of his now.
If I read the Forster (2011) study correctly it seems that he was able to replicate an effect (using designs characterized by weak power) across 17 consecutive studies. That alone should have been a huge red flag to reviewers and editors because it clearly fails the TIVA (Test of insufficient variance). Simply put across that many studies (with weak power) quite a few should have failed to find the hypothesized effect even if the effect was real.
I guess it has escaped Forster’s attention that the names of the authors of the report are printed on the first page of the report.
He refers to “the members of the commission who decided this” (procedure), not the statisticians writing the report
I can also see how the data presented in the Foerster, Epstude and Ozelsel (2009) paper would raise some red flags. For example, in study 1 the authors indicate that the 20 students who were primed with lust (by imagining sex with an attractive person) were largely unable to solve any of the pretty elementary creative insight task with 16 of the 20 participants scoring zero on the task and the other 4 only being able to solve one of the three questions (the only way that the mean and SD can be replicated). For study 2 the participants primed with love (using words flashed on a screen) were apparently unable to solve the GRE problems at better than chance while those primed with lust (also using words) were able to score three times as high.
“For example, in study 1 the authors indicate that the 20 students who were primed with lust (by imagining sex with an attractive person) were largely unable to solve any of the pretty elementary creative insight task with 16 of the 20 participants scoring zero on the task and the other 4 only being able to solve one of the three questions (the only way that the mean and SD can be replicated).”
Here is another paper in the same field with apparently almost all subjects giving exactly the same score, a different score according to different experimental conditions: http://www.sciencedirect.com/science/article/pii/S0022103111000345 “The effect of auditory versus visual violent media exposure on aggressive behaviour: The role of song lyrics, video clips and musical tone” by Heidi I. Brummert Lennings, Wayne A. Warburton. See Figure 1: https://www.dropbox.com/sh/l635y1ecwz24zcb/AABrC6qF8Q_DQM4ex7fNtWNwa?dl=0
What the…?
That’s odd. Table 1 is also odd, it is claimed to show raw data alongside data transformed with a log10 transform, but log10(3.83) is not .50 and log10(6.78) is not .60. Etc.
x=c(1,2,3,4,5)
log10(mean(x)) !=mean(log10(x))
Ohhh of course. Sorry.
PS dropbox link was wrong, here’s the good one:
https://www.dropbox.com/s/2539roj7k3np9jn/Hot%20chili%20sauce.png?dl=0
“Because I was sworn to secrecy with respect to the report and the email I received,” I couldn’t even read the first page!
Would you happen to have a link for the R code? I don’t really feel the need to defend Professor Foster, but in the statistical evaluation paper, it seems like they are using Fisher’s LSD method (maybe?) which is not recommended. See Multiple Comparisons: Theory and Methods by Jason Hsu. There is an adjustment that can be made, but without the code, I can’t tell if they made it.
Ed:
They are not using Fisher’s LSD in the report – they are using a finding of Fisher’s (Fisher, 1925, reference on page 101 of report) based on the fact that if X is a random variable with a uniform distribution, then -2logX has a chi-square distribution with 2 degrees of freedom. Thus a collection of independent p-values, which have a uniform distribution under the null hypothesis of no difference among groups under investigation, can be assessed by first converting them all ( -2(log(p)) ) and then summing them all up. The sum of N such values (the -2(log(p-values)) ) will have a chi-square distribution with 2N degrees of freedom. This is all discussed on page 98 of the report.
So, if too many p-values are near 0.0 or near 1.0 this non-uniform distribution can be quantitated by this “Fisher’s method” which in this case is not Fisher’s LSD, but rather this sum of -2logp quantities. A small p-value from this “Fisher’s method” shows that the collection of p-values is unlikely to have come from a uniform distribution, as should have happened when running a bunch of experiments with attendant random variation.
Since way too many of the tests for linear trend of results shown in the papers evaluated yield p-values near 1.0 (the results lined up in an increasing or decreasing fashion, lying nearly perfectly on a straight line) the results certainly appear suspicious, because random variability should have yielded some findings wherein trends across groups came out decidedly non-linear with smaller resultant test statistic p-values.
This is the conundrum for people who either practice sloppy research practices (sweeping awkward findings under the rug) or practice outright data fabrication. Collections of numbers have quantifiable distributional properties, and poor scientists who fall into the above categories do not have the chops to concoct collections of numbers that exhibit proper distributional characteristics. If you have the chops to fake such characteristics, you have the chops to be a proper, honest scientist. I recommend the latter approach for talented scientists – long term results are more rewarding. Sadly, incompetent folks will always be in our midst, and techniques such as those applied in this report will remain necessary in assessing scientific validity.
As an aside: Fisher used this method to show that Mendel’s pea experiments came out “too nice”. Mendel had been sweeping inconvenient plant breeding outcomes under the rug. Nonetheless, Mendel had so many results demonstrating trait heritability that his overall findings remained valid. We’ll see how things turn out in this case.
Well, my reference material is at the office and I’m at home, but I thought Fisher was wrong about Mendel’s work? I thought Mendel’s work was sort of the exception that makes the rule type of thing. My memory may be off on this. Haven’t read that stuff in a while.
One thing I haven’t understood about “collections of p-values” and similar discussions, is the effect of systematic bias or error in the experiments, or fabrications, whichever they may be. Most of us know about various biases, like “immortal time bias” in survival analysis or misclassification that is differential in nature. Hopefully I don’t appear to be an idiot with this comment, but shouldn’t systematic errors produce distributions that are more exponential or Poisson with over dispersion, within this context? And shouldn’t data fabrication produce the same appearance, as it likely results from systematic application of the fabrication process by the fabricator?
I thought for sure I just read a simulation paper evaluating systematic bias and given a vector X of covariates, the resulting estimates and their p-values took an exponential flavor in their distribution.
I could be wrong. Happens quite a bit.
Todd:
Many interesting papers have been written about the Fisher-Mendel controversy – several that I have read all reiterate several points:
– Mendel’s results were “too good”.
– Mendel was not intentionally committing fraud – he was running these experiments in the 1860s when data practices were far less advanced compared to modern practices. Mendel and / or assistants just didn’t appear to report results from some experiments that seemed odd. Overall, Mendel’s efforts are still awe-inspiring.
– Fisher’s findings were not inappropriate, though there are several alternate analytical / modeling strategies that the various authors argue better fit scenarios consistent with Mendel’s writings on the experimental procedures and data collection. Fisher himself admired Mendel’s work greatly, and was only pointing out that there were some problems with how Mendel reported findings, not that Mendel’s conclusions were inappropriate or that Mendel was intentionally attempting to mislead anyone. Fisher was interested in educating researchers as to proper data collection and assessment methods, and the Mendel materials presented a “teachable moment”. They still do.
Regarding the distributional properties of collections of p-values: Yes, if some bias is in play, then the distribution of p-values will deviate from a uniform distribution, so a sum of -2logp values will deviate from a chi-square distribution, so Fisher’s method will yield a small p-value. This is the outcome noted by the reviewers in the report. It is highly unlikely that in experiment after experiment, a test for linear trend will keep producing large p-values. Some kind of bias appears to be in play. The nature of that bias is what this whole controversy is about.
The R code has been distributed by the committee. I have it, if anyone is interested, email me.
Response to UvA report by Förster’s co-authors Nira Liberman, School of Psychological Sciences, Tel Aviv University, Israel and Markus Denzler, Federal University of Applied Administrative Sciences, Germany: https://dl.dropboxusercontent.com/u/133567/Generalresponse.pdf
Discussion on PubPeer, and links to R code etc https://pubpeer.com/publications/5439C6BFF5744F6F47A2E0E9456703
Interesting debate also here: http://rejectedpostsofdmayo.com/2015/06/09/fraudulent-until-proved-innocent-is-this-really-the-new-bayesian-forensics-rejected-post/comment-page-1/#comment-10723
Why does Retraction Watch not cover this discussion? It seems to be relevant for their previous report here
http://arxiv.org/abs/1506.07447 Fraud detection with statistics: A comment on “Evidential Value in ANOVA-Regression Results in Scientific Integrity Studies” (Klaassen, 2015) by Hannes Matuschek
Abstract: Klaassen in (Klaassen 2015) proposed a method for the detection of data manipulation given the means and standard deviations for the cells of a oneway ANOVA design. This comment critically reviews this method. In addition, inspired by this analysis, an alternative approach to test sample correlations over several experiments is derived. The results are in close agreement with the initial analysis reported by an anonymous whistleblower. Importantly, the statistic requires several similar experiments; a test for correlations between 3 sample means based on a single experiment must be considered as unreliable.
Peeters, Klaassen, van de Wiel respond to criticism on their report http://www.uva.nl/en/news-events/news/uva-news/content/news/2015/07/update-articles-jens-forster-investigated.html