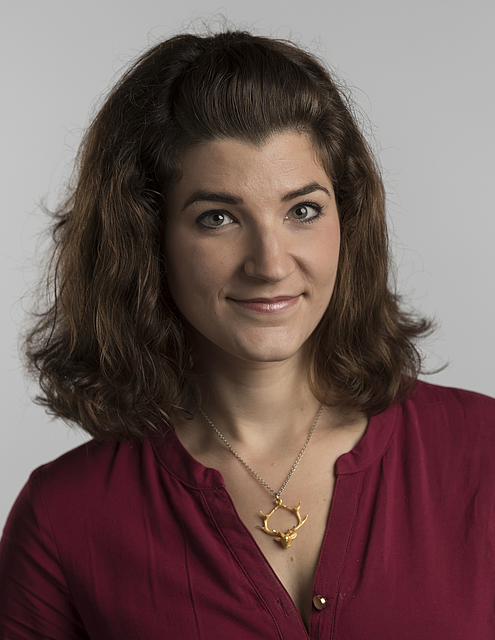
Two years ago, Julia Strand, an assistant professor of psychology at Carleton College, published a paper in Psychonomic Bulletin & Review about how people strain to listen in crowded spaces (think: when they’re doing the opposite of social distancing).
The article, titled “Talking points: A modulating circle reduces listening effort without improving speech recognition,” was a young scientist’s fantasy — splashy, fascinating findings in a well-known journal — and, according to Strand, it gave her fledgling career a jolt.
The data were “gorgeous,” she said, initially replicable and well-received:
We planned follow-up studies, started designing an app … for use in clinical settings, and I wrote and was awarded a National Institute of Health grant (my first!) to fund the work.
But — and, because this is Retraction Watch, you knew that was coming — all that changed. Writing in Medium recently, Strand described her dawning realization that her cherished study was deeply flawed (we urge you to read the whole post):
Several months later, we ran a follow-up study to replicate and extend the effect and were quite surprised that, under very similar conditions, the finding did not replicate. …
The difference was massive enough that I was confident it wasn’t just a fluke: you don’t go from 100% of participants showing an effect to 0% without something being systematically different.
That something turned out to be a coding error that, once corrected, nullified the results:
The effect that we thought we had discovered was just a programming bug.
The realization left Strand stunned:
When I identified the error, I was shocked. I felt physically ill. I had published something that was objectively, unquestionably wrong. I had celebrated this finding, presented it at conferences, published it, and gotten federal funding to keep studying it. And it was completely untrue.
She feared that once her colleagues learned of the “stupid mistake” they would not only disown her paper but they might disregard her as a scientist. She
worried that admitting the error and retracting the paper would jeopardize my job, my grant funding, and my professional reputation.
Strand, understandably, mulled what might happen if she kept quiet about her unfortunate discovery:
The bug was hard for me to identify; maybe no one else would ever find it. I could just go on with other research and nobody would ever know.
Obviously, I decided not to go that route.
Strand then ran down the list of “next-of-kin” to notify: co-authors, the journal, the NIH program officer overseeing her grant, her department, even a student who had used the data for her now-imperiled master’s defense:
The next day was the worst of my professional career. I spent all day emailing and calling to share the story of how I had screwed up. After doing that, part of me wanted to tell as few other people as possible. So why share this with an even wider audience?
However, she did spread the word, writing about the experience and posting about it on social media.
As far as we can tell, at least, the early returns are looking good. Strand’s decision to go public has received praise on Twitter.
David Folkenflik, a media correspondent for NPR, tweeted:
Lia Li, a researcher in London, wrote:
Strand writes:
The editor and publisher were understanding and ultimately opted not to retract the paper but to instead publish a revised version of the article, linked to from the original paper, with the results section updated to reflect the true (opposite) results. After spending months coming to terms with the fact that the paper would be retracted, it wasn’t.
The journal appended the following notice to both versions:
This paper is a corrected version of a previous manuscript published in 2018 (https://link.springer.com/article/10.3758/s13423-018-1489-7). While attempting to replicate and extend the original work, the first author discovered an error in the stimulus presentation program that invalidated the results. This paper presents the corrected results.
Strand tells Retraction Watch that the response to her self-lustration has been:
incredibly heartening. I didn’t know what kind of reaction this would get, and it has been great to see such support. I wanted to share the story so people could see that admitting a mistake doesn’t necessarily end your career; it’s an added benefit that now people can see the outpouring of support for transparency. This experience would have been less trying if I’d had models of what might happen and how the scientific community would respond. Although this has been a difficult experience, I’ll be very glad if it makes it easier for someone else to do the right thing in the future.
Like Retraction Watch? You can make a tax-deductible contribution to support our work, follow us on Twitter, like us on Facebook, add us to your RSS reader, or subscribe to our daily digest. If you find a retraction that’s not in our database, you can let us know here. For comments or feedback, email us at [email protected].
+10 points for “self-lustration”.
Thank you for posting.
This is the way things should be in science, and elsewhere, but isn’t always.
Very heartening.
Wishing Julia Strand, and all true scientists, well.
This could have happened to me. I has, but I was lucky enough to catch it in the very last minute. The way Strand dealt with it is what makes science superior to any other way of thinking.
What an enlightening post!
This is the way. You (Dr. Strand) will get support all over the world. As a researcher and teacher, we make all kinds of mistakes – big and small.
Sorry, I hate to be the Spielverderber here, but don’t people look at their data? The difference between 0 and 100% should be pretty clear without a computer.
This depends VERY MUCH on the complexity of the data being processed and/or the complexity of the processing itself. In my and many colleagues’ labs, any given task can easily generate tons of data even for a single participant, and everything then hinges on distilling valid indices from these data. I don’t know Dr. Strand’s paper and therefore cannot comment on her case specifically. But I know from research in my own lab that things can pretty quickly become very complex, and bugs are therefore sometimes hard to spot. One solution: Generate artificial probe raw datasets that simulate extreme cases. If they also show up as being extreme (and in the right direction) in whatever indices you derive from the data and plan to work with in a publication, then at least you have a modicum of certainty that your data processing script does what it supposed to do. Another solution: Have two different coders independently write the data analysis scripts and then compare whether the indices they arrive at are identical. If yes, then again you have that modicum of certainty.
This woman definitely did the right thing and I am not criticizing her. But before I went to grad school, I worked as what was then called a “computer programmer” in a local government agency for about three years. We were taught to check and test everything very carefully and keep detailed records. I was amazed when I got to grad school to see how cavalierly people tended to treat data and programming. They didn’t test their programs with dummy data and special conditions to see if the code worked correctly. They really paid little attention. There have been a fair number of instances where published papers by luminaries have had to be corrected or retracted because of a coding error, and in general the incorrect code in question has just been written by someone who is basically an amateur programmer, even though they may be highly skilled researchers in other ways.
https://www.nature.com/articles/467775a
Here’s an article from Nature about the problem of errors in code written by scientists who lack formal training in coding.
This act shows you have integrity, which is one of the most important traits to have as a human being (and a true scientist). You should be proud of yourself.
Thought experiment. What if a student/assistant discovered the error and pointed it out. I have done so early in the process and discovered opposite results. No problems. But if I had discovered it following publication and a grant, I’m sure I would have suffered.
Oliver C. Schultheiss, you may be right that this is not as transparent as it sounds, although in my understanding the data are not derived from test tubes but from observation of people. This limits the amount of data you have to process, I’d say.
But in any case, due dilligence requires some sort of check of codes, as we agree on, whether implementing parallel coding or test runs with dummy data. And the more complicated the data or the data analysis, the better the checks should be, obviously.
Read the post your are commenting on again. The issue was with the stimulus presentation code, not with the data analysis code. She could have triple (and maybe did) checked her data analysis code, ran it through dummy data or whatever and it would not have prevent anything in that case: the stimuli presented where not the one expected and thus generated different behavior.
Sorry, Olivier. I read in the post
‘That something turned out to be a coding error that, once corrected, nullified the results:’
I assumed this was in the data analysis part. It seems you are telling me the 100 to 0% problem was in the experimental design and execution.
That does not really invalidate my point, does it? Codes need to be tested, as any other part of an experiment does, whether they are used to generate data or analyze them. I hope we can agree on that.
I wonder if anybody can help with this:
(based on the info in this blog, I can’t access Medium from here, but the timeline seems clearly stated in this blog)
An experiment was done and published with an effect. Let’s call this the 100% effect. Later an experiment was performed with the same protocol. This gave 0 %. The difference was then traced to a coding error.
But if the coding error was in place during the execution and the analysis of both the 100% and the 0% experiment, why did the 0 % experiment not give the same 100% as the first experiment? Statistical fluctuations do not reproduce, of course, but this story is not one of statistical fluctuations.
If this timeline is correct, it seems to me there must have been another, unidentified error.
Agreed 🙂 Although testing for the right stimulus being output at the end of the chain seems hard. Let’s say it is about audio stimuli like it seems to be the case here, what to do ? Put a microphone and analyze the properties of the signal ? Maybe i am being a bit extreme but something unexpected can happen anywhere in the process (mixing up files, filtering in the audio chain that affect the signal, etc…) No easy job in my opinion