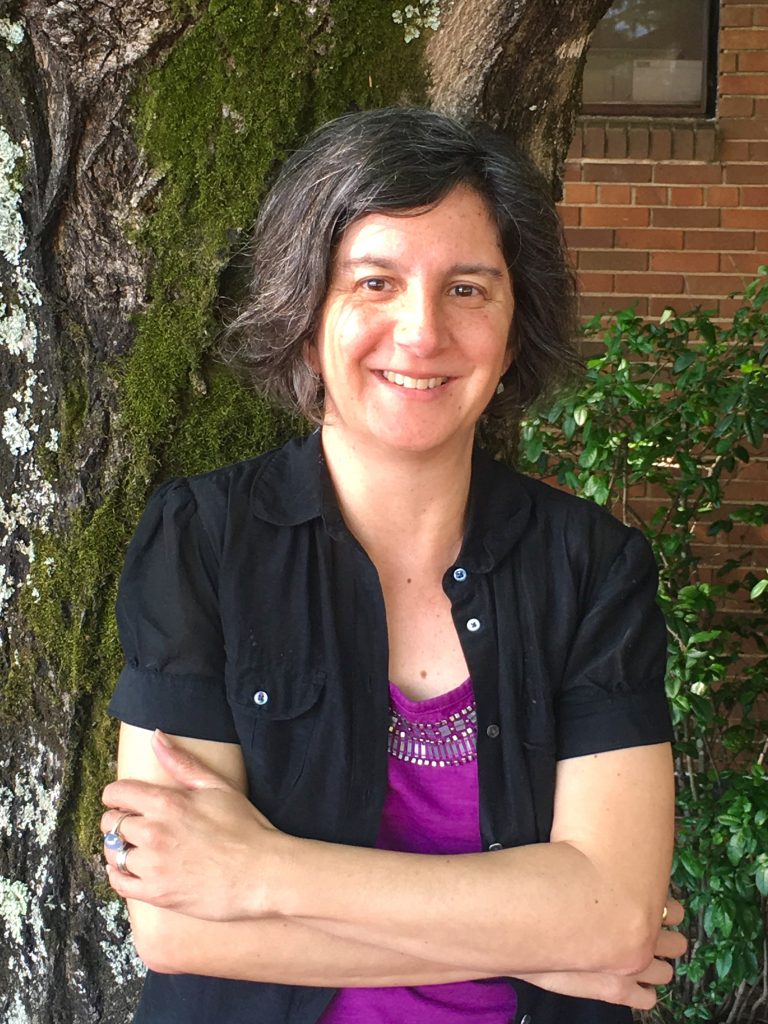
Three years ago, the American Statistical Association (ASA) expressed hope that the world would move to a “post-p-value era.” The statement in which they made that recommendation has been cited more than 1,700 times, and apparently, the organization has decided that era’s time has come. (At least one journal had already banned p values by 2016.) In an editorial in a special issue of The American Statistician out today, “Statistical Inference in the 21st Century: A World Beyond P<0.05,” the executive director of the ASA, Ron Wasserstein, along with two co-authors, recommends that when it comes to the term “statistically significant,” “don’t say it and don’t use it.” (More than 800 researchers signed onto a piece published in Nature yesterday calling for the same thing.) We asked Wasserstein’s co-author, Nicole Lazar of the University of Georgia, to answer a few questions about the move. Here are her responses, prepared in collaboration with Wasserstein and the editorial’s third co-author, Allen Schirm.
So the ASA wants to say goodbye to “statistically significant.” Why, and why now?
In the past few years there has been a growing recognition in the scientific and statistical communities that the standard ways of performing inference are not serving us well. This manifests itself in, for instance, the perceived crisis in science (of reproducibility, of credibility); increased publicity surrounding bad practices such as p-hacking (manipulating the data until statistical significance can be achieved); and perverse incentives especially in the academy that encourage “sexy” headline-grabbing results that may not have much substance in the long run. None of this is necessarily new, and indeed there are conversations in the statistics (and other) literature going back decades calling to abandon the language of statistical significance. The tone now is different, perhaps because of the more pervasive sense that what we’ve always done isn’t working, and so the time seemed opportune to renew the call.
Much of the editorial is an impassioned plea to embrace uncertainty. Can you explain?
The world is inherently an uncertain place. Our models of how it works — whether formal or informal, explicit or implicit — are often only crude approximations of reality. Likewise, our data about the world are subject to both random and systematic errors, even when collected with great care. So, our estimates are often highly uncertain; indeed, the p-value itself is uncertain. The bright-line thinking that is emblematic of declaring some results “statistically significant” (p<0.05) and others “not statistically significant” (p>0.05) obscures that uncertainty, and leads us to believe that our findings are on more solid ground than they actually are. We think that the time has come to fully acknowledge these facts and to adjust our statistical thinking accordingly.
Your editorial acknowledges that the Food and Drug Administration (FDA) “has long established drug review procedures that involve comparing p-values to significance thresholds for Phase III drug trials,” at least in part because it wants to “avoid turning every drug decision into a court battle.” Isn’t there a risk that ending the use of statistical significance will empower those who use weak science to approve drugs that don’t work, or are dangerous?
We don’t think so. All of the science is still there — the biomedical expertise, the carefully designed and executed experiments, the data, the effect sizes, the measures of uncertainty are all still there. Researchers can still compute summaries such as p-values (just don’t use a threshold) or Bayesian measures (ditto). Product developers would still need to make a convincing case for efficacy. Eliminating statistical significance does not mean that “anything goes.” The expectation is that the FDA would develop new standards that don’t depend on a single metric, but rather take into account the full set of measured results.
Furthermore, as we have seen in many other contexts, relying on statistical significance alone often results in weak science. While the FDA has taken a conservative stance about the evidence needed to declare a new drug effective, which is understandable, that comes with a cost. Namely, drugs that might be effective according to better measures of evidence are potentially rejected.
Tell us about some of the other 43 articles in the issue.
The issue includes, we think, something for everyone. It represents the diversity of opinion that we within the statistical community hold. Importantly, we don’t think that there is one sure-fire solution for every situation. In the Special Issue, there are papers that call for retaining p-values in some form or other, but changing how they are used; other papers propose alternatives to p-values; others still advocate more radical approaches to the questions of statistical inference. We don’t claim at this stage to have “the answer.” Rather, the papers in the issue are an attempt to start a deeper conversation about the best ways forward for science and statistics. For that reason we also have some articles on how to change the landscape, starting with how we train students at all levels, and culminating with alternative publication models such as preregistered reports and changes to editorial practices at journals.
Anything else you’d like to add?
While some of the changes proposed in the Special Issue will take time to sort out and implement, the abandonment of statistical significance – and, for example, declarations that there is an effect or there is not an effect – should start right away. That alone will be an improvement in practice that will spur further improvements. But it’s not enough to abandon statistical significance based on categorizing p-values. One similarly should not categorize other statistical measures, such as confidence intervals and Bayes factors. Categorization and categorical thinking are the fundamental problems, not the p-value in and of itself.
We’d also like to emphasize that there is not now, and likely never will be, one solution that fits all situations. Certainly automated procedures for data analysis that are sometimes put forth are not the answer. Rather, the solutions to the problems highlighted in the original ASA statement are to be found in adherence to sound principles, not in the use of specific methods and tools.
One such principle about which there has been contentious debate, especially in the Frequentist versus Bayesian wars, is objectivity. It is important to understand and accept that while objectivity should be the goal of scientific research, pure objectivity can never be achieved. Science entails intrinsically subjective decisions, and expert judgment – applied with as much objectivity and as little bias as possible – is essential to sound science.
Finally, reach out to your colleagues in other fields, other sectors, and other professions. Collaboration and collective action are needed if we really are going to effect change.
Like Retraction Watch? You can make a tax-deductible contribution to support our growth, follow us on Twitter, like us on Facebook, add us to your RSS reader, sign up for an email every time there’s a new post (look for the “follow” button at the lower right part of your screen), or subscribe to our daily digest. If you find a retraction that’s not in our database, you can let us know here. For comments or feedback, email us at [email protected].
Why not unpack p=0.05 and say something like, “The likelihood that the difference in remission rates that we report herein had no causal association with whether patients received Drug A or placebo is approximately equivalent to that of being dealt two pairs in a hand of stud poker?” Substitute analogy, with its inherently greater specificity of diverse verbs and predicates, for repetitive adjectival labeling, pairing the most precise of many analogies adapted to most appropriate subreaderships, pursuant to predetermined, well thought-out “inertias of credulity?”
Five card or seven card?
I agree, I know in my own dissertation that my data had marginal correlations, however the p values were high??? Obviously more data needed to be gathered in those areas. In addition we all know that correlations obviously do not prove causation, but assigning p values to correlations somehow assigns an aura of higher level of predictability than is deserved.
John
Dr. John Holliday
623-236-0272
[email protected]
Dude, you are misinterpreting the correlation test. What ever your correlation it tests whether samples size is sufficient to conclude the coefficient or not.
Hi there,
I am still unable to understand that if these guys stopped saying statistical significance then what is alternative to it?
What interests are there? As I know the bayesian vs frequentist conflicts.
But Without new theory we can’t discard older one.
Any data follows some probability distribution. It is described by central nature and variable nature. So both accounts, certainity and uncertainty are considered here. If some other process results(data) influenceing on study variable regularly then data must reflect change in location (central nature) or variability or both, this is called significance.
For example, hight depends on gender. Or gender influences hight. So we can’t build a single model of distribution. We need two different models to discuss on hight. If sample size is smaller than desired it may miss significance. But once it saying significance then it is significant, if all methods of data collection are rational and appropriate test is used.
Now if a drug is not useful and some is useful, how to decide it? There must be objectives process to convince evidences. And if you what to believe in intuitions and experience then why we need science too? And these scientist too?
We must understand science is not opinion. It is searching of truth and convincing findings to world for betterment for knowledge, so we could control our living and surrounding (universe).
Your truth and my truth may differ but scientific truth should not if conditions are identical. If it differ you need to give that what condition are not identical, causing difference.
Reproducibility is responsibility of scientist not statisticians. Scientist arrange studies conveniently and blaming to statistics for failure, is it acceptable?
The arguments against significance are like (in post p-val era) ” I gave a medicine arbitrary from dispensary, and my intuitions says patient body temperature will be normal in next few years”.
In place of blaming wrong practice they are blaming statistics. God bless them.