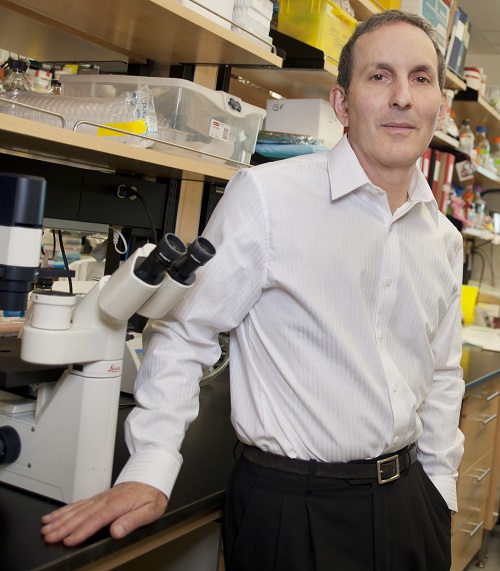
By all accounts, science is facing a crisis: Too many preclinical studies aren’t reproducible, leading to wasted time and effort by researchers around the world. Today in Cell Metabolism, Daniel Drucker at the Lunenfeld-Tanenbaum Research Institute of Mount Sinai Hospital in Toronto details numerous ways to make this early research more robust. His most important advice: more transparent reporting of all results (not just the positive findings), along with quantifying, reporting, tracking, and rewarding reproducibility, for both scientists and journals and universities/research institutes.
Retraction Watch: Which of your recommendations will researchers most object to, and why?
Daniel Drucker: Most folks won’t like comprehensive reporting of negative data (will spoil many a clean, exciting, yet perhaps somewhat narrow story), and many will rightfully point to the difficulty in the ascertainment of and precise definition of reproducibility.
RW: You tell an interesting story of how your own lab couldn’t reproduce some of your most exciting observations in mice after simply moving your lab across the street. What was the explanation for the discrepancy, and what lessons did you learn from it?
DD: Animal facility environments are often very different. Housing conditions, bedding, cages, noise, water, food, lights on and off cycles and the resident microbiome differ across many facilities, and each one of these factors can impact experimental results.
RW: One of your recommendations is to apply the same rules of human clinical trials to preclinical research – as in, don’t discard outliers, report all data, etc. (Although critics of clinical trials argue not all data are reported, either.) What will be the main challenges in implementing this practice, and how can they be addressed?
DD: There are many challenges, including our culture of accentuating and rewarding the positive, exciting, yet perhaps restricted story, and sometimes unintentionally penalizing (by reduction of enthusiasm) the more transparent reporting of all data — including all of the negative data that may not support (or may even refute) a key hypothesis. However, more complete transparent reporting need not be arduous. If one orders and uses 1,000 mice a year, reporting what was done to the mice, and what the results were, should not be so difficult. Let’s start slowly, perhaps reporting first to our own Institutional Review Boards (IRBs) and institutions. Then let’s see if we should make this reporting more publicly available, including to our colleagues in academia, industry, and journals, etc.
RW: In your lab, you ask researchers to reproduce a finding in multiple animal models before examining whether a supporting mechanism is evident ex vivo in cell culture, as well. This is the opposite strategy many preclinical researchers use – why do you do it? How do you compensate for the extra time and effort involved?
DD: I approach most problems first from my background as a clinician. I am used to thinking about disease processes and therapeutic approaches in the context of a living human, then in a whole animal. Clinical relevance and therapeutic potential are much more challenging if we start from isolated cells or biochemistry, which has not yet been first validated in whole animal models. That does not mean my strategy is correct, preferable, or the best way to proceed in each instance, it just reflects the importance with which I personally perceive and value whole animal and human physiology and pathophysiology.
RW: I think most scientists are now aware of problems related to misidentified cell lines, but you raise another important issue – the continued use of non-validated antibodies, which can have a major impact on preclinical experiments. Why do you think the community hasn’t caught onto that as a major problem yet? What can be done about it?
The antibody problem is very familiar to most scientists and ideally should be quite simple to address with positive and negative controls. Yet this validation process is a bit cumbersome and time consuming, and many folks just don’t bother; they trust by default an off-the-shelf reagent. Either some folks have not been taught to routinely validate reagents, or they are too busy, or they are not aware that many reagents available are imperfect and might contribute to misleading results. It usually takes at least one instance of generating suboptimal data with a flawed antibody for the sobering lesson to sink in.
Like Retraction Watch? Consider making a tax-deductible contribution to support our growth. You can also follow us on Twitter, like us on Facebook, add us to your RSS reader, sign up on our homepage for an email every time there’s a new post, or subscribe to our daily digest. Click here to review our Comments Policy. For a sneak peek at what we’re working on, click here.