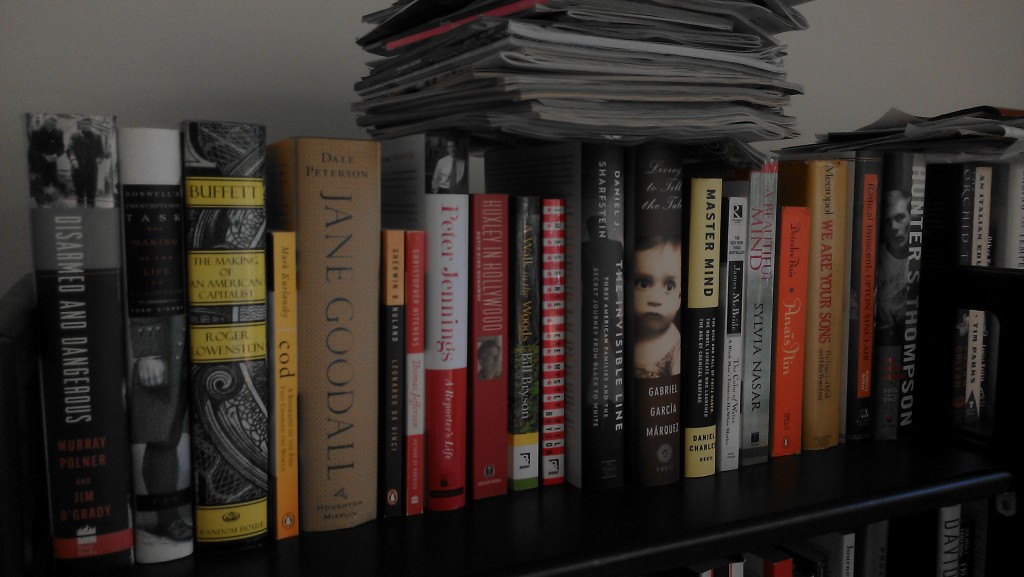
Before we present this week’s Weekend Reads, a question: Do you enjoy our weekly roundup? If so, we could really use your help. Would you consider a tax-deductible donation to support Weekend Reads, and our daily work? Thanks in advance.
The week at Retraction Watch featured:
- An Elsevier book chapter that claims COVID-19 came from space;
- An Elsevier journal that shares concerns about a ‘transparently ridiculous’ genetics paper
- A paper retracted because the references didn’t say what the authors said they did — and because the authors couldn’t come up with the underlying data
- An investigation by Arizona State into two of its former neuroscience researchers
Our list of retracted or withdrawn COVID-19 papers is up to 32.
Here’s what was happening elsewhere:
- “It’s like if you throw a dice and you get exactly the same sequence of numbers several times.” On Russian vaccine data.
- “More than a month after the Indian drug regulator’s controversial approval of Biocon’s drug Itolizumab, new documents and the company’s own admissions raise serious doubts about the quality of the clinical trial Biocon conducted.”
- In “[A] COVID-19 related preprint – that quickly disappeared and reappeared after being discussed on social media,” a “Hollywood doctor treats COVID-19 patients with his brother’s miracle cocktail,” Elisabeth Bik writes.
- “There will be no p-values in any paper that I co-author in the next 12 months.” Will you take the p-value pledge?
- A medical society removed Deepak Chopra as a keynoter after a backlash.
- “On having no good options: Why I removed my name from a paper.”
- “The Trump adviser weighed in on Fauci’s planned responses to outlets including Bloomberg News, BuzzFeed, Huffington Post and the science journal Cell.”
- “[I] believe that PubPeer has appealing features, but has the potential to do harm to innocent authors, or to be used for personal attacks.”
- “Can we estimate a monetary value of scientific publications?”
- “Fraud by Numbers:”… “In the age of metrics, quantity trumps quality even in fraud,” says one observer.
- “One in four economics preprints ‘fail to end up in a journal.’”
- “COVID-19 Blamed for Weaker Research Published by Top-Tier Journals in 2020.”
- “Unconsented acknowledgments as a form of authorship abuse: What can be done about it?” asks a new paper.
- Two researchers appeal for empowering a culture of failure in science.
- About one in 20 Australian ecology academics “have had their work ‘unduly modified’ by employers, a study suggests.”
- “Dhaka University authorities have found proof of plagiarism in a joint article authored by two of its teachers.”
- “How reliable and useful is Cabell’s Blacklist [of predatory journals]? A data-driven analysis.”
- “A qualitative content analysis of watchlists vs safelists: How do they address the issue of predatory publishing?”
- James Heathers digs out an old unpublished blog post about Brian Wansink, the former Cornell food marketing researcher with 18 retractions.
- Given the backdrop of the COVID-19 pandemic, “we need to think about providing guard rails to viral science to advance the goals of robustness and reproducibility while disseminating research.”
- “Polarisation has often occurred before deliberation, but that same polarisation has been good for the research altmetrics.”
Like Retraction Watch? You can make a tax-deductible contribution to support our work, follow us on Twitter, like us on Facebook, add us to your RSS reader, or subscribe to our daily digest. If you find a retraction that’s not in our database, you can let us know here. For comments or feedback, email us at [email protected].
“About one in 20 Australian ecology academics “have had their work ‘unduly modified’ by employers, a study suggests.” ”
Anyone have a non-paywalled source of this study? Thanks in advance.
The paper itself is open access: https://doi.org/10.1111/conl.12757
Thanks Finder!
The RW lead in didn’t give enough to search on.
RE: culture of failure
Philosophically, that makes great sense and I agree. Practically, failure is very difficult to prove. Here’s a devil’s advocate scenario: take the hypothesis X affects Y. How many conditions and how many experimental approaches must one try before definitively saying it doesn’t? If you’re interested in what affects Y, the most efficient way to approach it is not to definitively, publishably (whatever that means) nail down whether or not X does, but to screen the whole alphabet in a few carefully chosen assays, and follow up on whatever you find DOES affect Y.