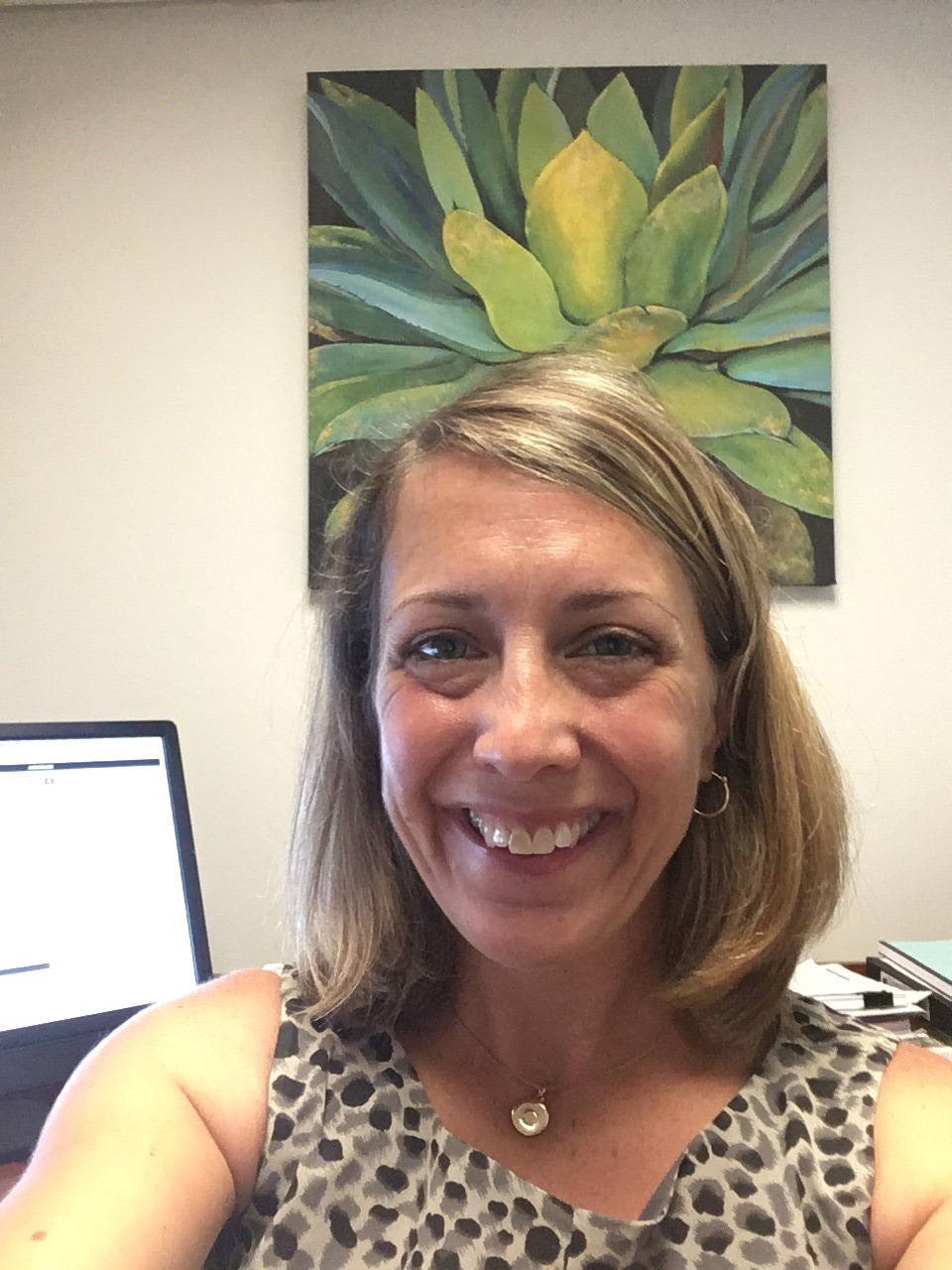
One of the most common reasons for retractions is image manipulation. When searching for evidence of it, researchers often rely on what their eyes tell them. But what if screening tools could help? Last week, researchers described a new automated tool to screen images for duplication (reported by Nature News); with help from publishing giant Elsevier, another group at Harvard Medical School is developing a different approach. We spoke with creators Mary Walsh, Chief Scientific Investigator in the Office for Professional Standards and Integrity, and Daniel Wainstock, Associate Director of Research Integrity, about how the tool works, and why — unlike the other recently described automated tool — they want to make theirs freely available.
Retraction Watch: What prompted you to develop this tool?
Mary Walsh and Daniel Wainstock: When reviewing concerns that two published images, representing the results of different experiments, might actually be the same, we typically assess whether the images are too similar to derive from different samples. The answer is often obvious to the naked eye, but not always, and we wanted to determine if it was possible to quantify the similarities.
Tools like the ones provided by the Office of Research Integrity in the US Department of Health and Human Services are qualitative – they highlight certain types of similarities and differences. But we were interested in quantitative information (e.g., a similarity score), so we initiated a collaboration with the Harvard Medical School (HMS) Image and Data Analysis Core (IDAC) facility to develop these tools.
RW: We know other people and journals screen images to look for manipulation. How is your technology different, and why is it necessary?
MW and DW: That kind of approach is driven by expert analysis, and is absolutely essential, to understand the significance of manipulation or image duplication. Our new tools should ideally be used before those experts get involved, to do two things: (A) screen through a large number of images so that the experts can concentrate on the ones that require attention, and (B) quantify similarities between images, so experts can benefit from statistical analyses in their assessment. (Work from Acuna et al. has just recently described a different approach to screening images.)
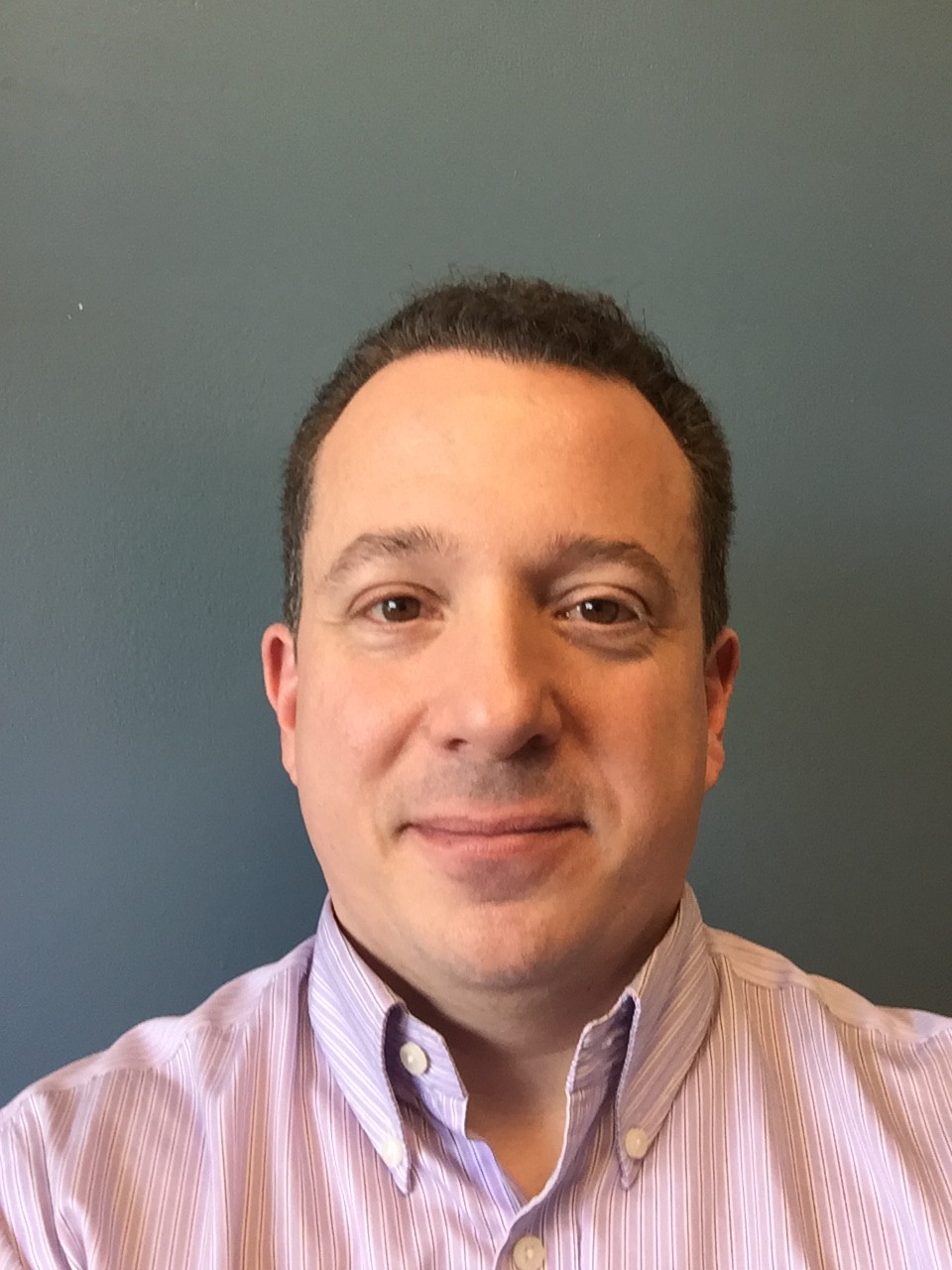
RW: How do these tools detect image duplications and manipulations?
MW and DW: Our first tool quantifies the correlation between two images. A user who wants to compare two images plugs them into the tool, and defines three or four points of similarity between them that serve as anchoring points for comparisons. The tool aligns the images and gives the user both a picture of the overlap between them and a quantitation of their correlation. This first tool has other features, such as an “intensity inspector” that identifies regions within images that are highly uniform in intensity. Given the natural intensity variations throughout most microscopy images of biological samples, very high uniformity may indicate that the image was manually edited or processed. There are benign explanations for many irregularities identified by the tool, but the analysis helps identify areas for more in-depth study.
Our second tool minimizes user input and may eventually allow automated screening (e.g., to determine whether a given image has actually been published before in another form). This tool takes a machine-learning approach using Siamese neural networks. Our collaborators at IDAC created a synthetic library of images altered in ways typical of published biological images (i.e., they cropped, resized, and labeled them, etc.). They then defined all the altered versions of each starting image as the “same” as the image it came from, defined all the different starting images as “different” from each other, and trained the network on this large set of positive (“same”) and negative (“different”) control images. Two new images can now be inputted into the computer, and the network will output a probability that they are the “same” (a duplicated image) or “different” (similar but distinct), based on its training.
However, “same” does not mean identical. Pixel-by-pixel comparisons detect identical regions of images, and they’re very useful, but they may not detect when the same image has been reused in a different way (e.g., saved at a lower resolution, or rescanned), so our second tool is complementary to the pixel-by-pixel methods that people have previously published.
RW: Do you envision this as a tool primarily for researchers before they submit manuscripts, or for journals when vetting submissions?
MW and DW: All of the above. The first tool could also be used for teaching. For example, in a fluorescence microscopy image, the background may look like it’s just a uniform black, but it’s actually got a lot of variation or background noise in it, and many quantitative studies make use of that background noise. Someone prepping that image for publication (e.g., a trainee) might adjust the image contrast a little too much, erasing much of the background noise. The “intensity inspector” tool would point that out and give researchers the opportunity to talk about best practices in their particular scientific field. These kinds of features may also help journals checking for image manipulation. And of course the screening tool would be useful to any researcher or journal trying to ensure that inappropriately duplicated images don’t wind up in published articles.
RW: Can you say how much financial support Elsevier is contributing to the project? Given that they are supporting it, will other researchers and/or publishers be able to use it, as well?
MW and DW: The work is driven by our office and the IDAC group; the quantitation tool was developed before Elsevier became involved. Once we started thinking about the screening tool, our collaborators at the IDAC facility told us they would need additional funding for the project so they could devote more time to it. Since our budget didn’t have the necessary flexibility at the time, Elsevier made a small gift to keep the project moving. Of course, we all agree that these tools need to be open source, so that anyone and everyone can test, use, improve, and build on them. We have taken the first steps in that direction via GitHub.
RW: When will a version of the tool be available to the community, do you expect?
MW and DW: We’ve posted a pre-print manuscript about the second tool, which lays the groundwork for screening. We hope to post at least one more pre-print manuscript, about the quantitation tool, later this year, and will continue to post on our methods, the source code, and the results we’ve obtained with them. But that’s just the beginning of the process of developing usable tools. The community will define how best to train a screening tool, and how to interpret the outputs of these tools, so they can be integrated into the qualitative toolkits that experts use every day. We’ll also need large numbers of examples of duplicated images to test and benchmark these tools…. So if your retractiondatabase.org [RW’s database of retractions] (or an analogous project at the HEADT Centre [which gathers images from retracted publications]) eventually provides public access to a pipeline of the specific images that drive retractions, that kind of resource would contribute to the development process, too!
Like Retraction Watch? You can make a tax-deductible contribution to support our growth, follow us on Twitter, like us on Facebook, add us to your RSS reader, sign up for an email every time there’s a new post (look for the “follow” button at the lower right part of your screen), or subscribe to our daily digest. If you find a retraction that’s not in our database, you can let us know here. For comments or feedback, email us at [email protected].
This is a welcome development, but in my humble opinion the post-publication peer review is becoming a distraction when it is too focused on image doctoring, rather than addressing conceptual fallacies in published articles.
Realism and empiricism come before philosophy and intellectualism unless you are in France.
Huh? How is a doctored image not a conceptual fallacy? Images are primary data upon which the findings of the published article are purportedly based.
Now if only Nature and Elsevier would get serious about at least issuing expressions of concern for problematic papers with image issues.
https://pubpeer.com/publications/BBC278257761C0C12E5D38844873DF
https://pubpeer.com/publications/F8EAE6A87C846AE0E114DF3C159CC6
https://pubpeer.com/publications/8716105A692122E6AD4DBD2480B1F8
Is there a reason you highlighted Nature and Elsevier, but have a first link that goes to Oncogene?
Ocogene – © 2018 Macmillan Publishers Limited, part of Springer Nature. All rights reserved.
Not every “image manipulation” is a conceptual fallacy. What I meant, however, was that image manipulation is seen as an easy target to detect fraud, while more serious problems such as inappropriate use of experimental models or statements not supported by any data or references may still go unnoticed.
The pre-print publication link doesn’t work. Can you please re-post?
It had an extra character at the end of it. Fixed, thanks for flagging.
Just so there’s no confusion, our group has been called the HMS Office for Professional Standards and Integrity, but we can now be found as part of a more integrated whole at the HMS Office for Academic and Research Integrity: https://ari.hms.harvard.edu/