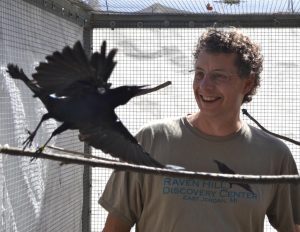
Not every study contains accurate information — but over time, some of those incorrect findings can become canonized as “fact.” How does this happen? And how can we avoid its impact on the scientific research? Author of a study published on arXiv in September, Carl Bergstrom from the University of Washington in Seattle, explains how the fight over information is like a rugby match, with competing sides pushing the ball towards fact or falsehood — and how to help ensure the ball moves in the right direction.
Retraction Watch: What factors play a role in making false statements seem true?
Carl Bergstrom: There are two phenomena at the core of the problem. The first is that experiments sometimes generate false positive results. The second is publication bias: the tendency of authors to preferentially write up and journals to preferentially publish “positive” results, such as statistically significant differences between groups or treatments, correlations between variables, or rejections of a null hypothesis. Publication bias makes it difficult for readers to evaluate the literature properly. In principle, a reader who is alert to the problem might be able to adjust for this bias in her evaluation of the literature, but she needs to make an active effort to do so and she needs to know the extent to which publication bias pervades the literature. This we seldom know.
Taken together, false positives and publication bias have the potential to cause serious trouble. Theodore Sterling noticed this over a half-century ago (T. Sterling 1959 J. Am. Stat. Assoc. 5:430–34) and John Ioannidis brought it to the attention of the biomedical research community in his 2005 PLOS Medicine paper “Why Most Published Research Findings Are False.” The gist of the problem is a basic application of Bayes’ rule: If true positives are rare and false positives are not uncommon, most published positives will be false positives. If in addition most published studies are positive, a high fraction of the published literature will be false.
In our paper, we do for facts what John did for individual experimental findings. By facts, we are referring to claims that are supported by multiple lines of evidence from multiple experiments, such as “A protein called Dicer initiates the eukaryotic RNAi pathway by slicing dsRNAs into small fragments.” The problem is that if many individual studies are likely to be false, perhaps some of the claims we accept as facts are false as well. We model how the community’s confidence in a claim shifts as successive results are published. The sociologist Bruno Latour famously compared this process to the dynamics of a rugby ball pushed alternatively toward fact or falsehood by two competing teams. We provide a formal model of how the ball is driven up and down the epistemological pitch until one of the goal lines is reached and a claim is canonized as fact or rejected as false.
RW: You told us in an email about the study that “unless a sufficiently high fraction of negative results are published—typically on the order of 20 to 30 percent—many false claims will inappropriately be established as fact.” How did you arrive at this figure?
CB: The 20 to 30 percent figure is a ballpark range for where things begin to go wrong in our model. There will be factors in the real world that we have not incorporated, and our choices of parameter ranges might not be exactly right. Thus our aim is not to make an exact estimate of what this number is for any particular field, but rather to highlight the mechanistic processes that lead false claims to become established as facts. The important take-home here is just the order of magnitude: Whether 20%, 30%, or 40%, it’s pretty clear that you don’t have to publish 95% of your negative results, but you also can’t get away with publishing only 5% of them.
RW: What would you estimate is the current rate of negative results?
CB: The problem is that we really have no idea. We can measure the fraction of published results that are negative: One study found that in most fields only about 20% of the published results, and in some fields such as psychology and ecology, fewer than 10% of the published results are negative (Fanelli 2012 Scientometrics 90:891–904). But what we really need to know is the converse: the fraction of negative results that are actually published.
The best available evidence comes from registered clinical trials. There, the situation looks to be pretty dire in some cases. Take a 2008 meta-analysis of 74 FDA-registered studies of antidepressants (Turner et al 2008 N Engl J Med 358:252-260). In that analysis, 37 of 38 positive studies were published, but only 3 of 36 negative studies were. Negative studies were published at less than 10% the rate of positive studies, and puts us squarely within the domain where false claims can be readily canonized as fact.
RW: How do systematic reviews and meta-analyses influence the spread of false statements?
CB: We haven’t taken systematic reviews and meta-analyses into account in our model; we’re looking a primary literature only. But you can imagine these would be important arbiters of “facthood” and that the issues that John Ioannidis has recently brought to light could exacerbate the problem.
RW: How can researchers combat the spread of false “facts”?
CB: As authors, design your studies so that negative results will be meaningful and sufficiently powered should they occur. Then publish your results, irrespective of the outcome. As reviewers and editors, recognize the importance of negative results to a properly functioning scientific community. As members of hiring, tenure, and promotion committees, don’t penalize researchers who take the time to publish the negative findings that our fields so badly require.
RW: Anything else you’d like to add?
CB: Yes. We’ve already seen science denialists on both ends of the ideological spectrum appealing to our results in efforts to discredit the science of whatever it is they are opposed to. For example, one prominent right-wing think tank issued a press release that tried to use our work, together with John’s meta-analysis work discussed above, to cast doubt upon the fact of anthropogenic climate change. This is a gross misrepresentation of our findings. The facts that science denialists target are almost always very different from the types of facts we are modeling. We are modeling small-scale facts of modest import, the kind that would be established based on one or two dozen studies and then considered settled. The reality of anthropogenic climate change, the lack of connection between vaccination and autism, or the causative role of smoking in cancer are very different. Facts of this sort have enormous practical importance; they are supported by massive volumes of research; and they have been established despite well-funded groups with powerful incentives to expose any evidence that might give cause for skepticism. The process by which false claims can become canonized as fact in our model simply would not operate under these circumstances.
This brings me to a broader point. I am definitely not a pessimist about the state of science, or about its ability to construct useful representations of the universe we inhabit. I think that science is an extremely effective way to learn about the physical world. While I do not believe that science is perfect, it should be obvious that by using mathematical and statistical models to illuminate the places where science could be better, we are working to improve science, not de-legitimize it.
Like Retraction Watch? Consider making a tax-deductible contribution to support our growth. You can also follow us on Twitter, like us on Facebook, add us to your RSS reader, sign up on our homepage for an email every time there’s a new post, or subscribe to our daily digest. Click here to review our Comments Policy. For a sneak peek at what we’re working on, click here.
Does no one teach regression to the mean anymore? Any surprising result is likely to be do to outliers, and hence a retest will “move the result toward the mean”. This is hardly new.