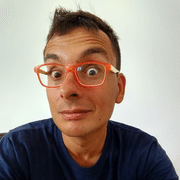
Retraction Watch readers may recall that in 2014, publisher Springer and IEEE were forced to retract more than 120 conference proceedings because the papers were all fakes, written by the devilishly clever SCIgen program and somehow published after peer review. So perhaps it was inevitable that fake computer-generated peer reviews were next.
In a chapter called “Your Paper has been Accepted, Rejected, or Whatever: Automatic Generation of Scientific Paper Reviews,” a group of researchers at the University of Trieste “investigate the feasibility of a tool capable of generating fake reviews for a given scientific paper automatically.” And 30% of the time, people couldn’t tell the difference. “While a tool of this kind cannot possibly deceive any rigorous editorial procedure,” the authors conclude, “it could nevertheless find a role in several questionable scenarios and magnify the scale of scholarly frauds.”
We spoke to one of the chapter’s authors, Eric Medvet, by email.
Retraction Watch: In the paper, you test the feasibility of computer-generated fake peer reviews. Why?
Eric Medvet: We were inspired by the case of SCIgen, a “nonsense Computer Science papers generator” which was developed in 2005 by a MIT team and released as a web application. Despite the fact that the motivation of SCIgen authors was no more than a joke about bogus journals and conferences, the impact of SCIgen of the scholarly publishing system has been disruptive. A recent article in The Guardian well summarizes the impact of SCIgen in its tilte: “How computer-generated fake papers are flooding academia.” We think that this impact is the result of a combination of pressure (“publish or perish”), incentives (research evaluation shifting to quantity rather than quality), opportunities (predatory journals and conferences), misconduct, and, finally, the availability of the SCIgen tool. Similar ingredients exist in peer review, but a tool is missing. So, what if such a tool existed? Is such a tool feasible? That was our motivation.
RW: You note that there are many people who would be interested in a tool that can generate fake reviews for free – who, specifically? And now that the tool has been created, are you afraid it might fall into the wrong hands?
EM: We imagine that at least two categories of subjects may be interested in generating many (fake) reviews at no cost: Scholars who want to take part in many program committees or editorial boards, just to earn the corresponding credits without actually spending time in reviewing papers; or predatory journals interested in inflating their credibility by sending many reviews to authors. In both cases, we are thinking about subjects who commit misconduct.
We did not release our tool publicly, however we think that it could easily re-implemented and possibly improved by any practitioner with the right skills. We hope, however, that the impact of our work will be more in the form of an argument about the ever increasing debate about the role of peer review and, more in general, about the scholarly publishing system.
RW: Can you explain in basic terms (ie, for people with little to no understanding of computer programming) how the tool is able to generate fake reviews? Do you decide ahead of time whether the paper should get a recommendation of “accept, neutral, or reject” then use the program to write a review around that?
EM: In brief, our tool is a black box in which you input the paper to be reviewed and the desired recommendation (one among accept, reject, or neutral). The tool builds the fake review by performing many processing steps, all aiming at producing a piece of text which meets the following three requirements: It appears as human-written, it appears as specific for the input paper, and it expresses the desired recommendation.
Internally, the tool bases on many techniques of the Natural Language Processing research field, including sentiment analysis, part-of-speech tagging, and named entity recognition.
RW: You show the computer generated reviews to 16 people – including 8 who were very familiar with science publishing – along with other reviews created by humans, available via journals that make them public (such as eLife) and reviews your lab has received. Amazingly, it seemed like one out of three fake reviews looked genuine to human reviewers. Did that surprise you?
EM: Indeed we were surprised by this result, also because our tool is definitely not so complicated in its inner workings. However, the fact that the generated reviews are built by assembling together pieces of real reviews makes this figure sound. If, by chance, a generated review combines sentences which are not specific, but credible, the review itself may appear as written by a real, human reviewer even to the eyes of an experienced reader.
RW: In a separate experiment involving 13 people (7 experienced), you asked them to note which reviews influenced their opinion most about a paper. Here, there were more surprising findings – 25% of the time, an experienced reviewer disagreed with the real review and agreed with the fake one. And one-quarter of the time, people said they were most influenced by the fake review. Again, how did you react to those findings?
EM: That’s probably the most interesting result of our work. In practice, it looks like that a fake review which is injected in a peer review process is able to manipulate the outcome of the process: however, you should note that the scale of our experimentation is quite small and that by no means it is an accurate replica of a full discussion between reviewers. An explanation for such a surprising result, anyway, is in the fact that our subjects were required to take a decision (accept or reject); they were not allowed to say “something here does not sound correct, I’ll not decide.” By the way, when, for instance, a real program chair of a conference is facing many web forms like the one we showed to our subjects, he/she is in the same situation: He/she has to take a decision. He/she could even decide without actually reading the reviews, or maybe by giving them just a shallow read. And that’s exactly the point! If you read a SCIgen paper, it is quite easy to spot the fact that it’s not sound. But if you do not read it…
RW: These findings suggest that an entirely fake review could subvert the peer-review process, by edging out the genuine reviews in editors’ decision-making. Does that seem dangerous to you?
EM: To me, “dangerous” is probably too much. But, of course, the scientific community needs to think (and is already thinking) about peer review and, more in general, scholarly publishing. From another point of view: the scale of the system is becoming larger and larger. Assuming that everyone is behaving correctly is probably not a wise thing.
Like Retraction Watch? Consider making a tax-deductible contribution to support our growth. You can also follow us on Twitter, like us on Facebook, add us to your RSS reader, sign up on our homepage for an email every time there’s a new post, or subscribe to our daily digest. Click here to review our Comments Policy. For a sneak peek at what we’re working on, click here.
Ah, the seething spawn of perverse incentives!
Been to your doctor lately and watched him/her inputting billing codes into an EHR so his/her healthcare system can optimize (inflate) the surrogate indicators of the value of your visit to maximize payment while your problems are given short shrift?
Same set of problems scaled up and running full tilt.
Good heavens.
How lazy have full professors become? Or how intent on publishing the unpublishable have journals become? I don’t know which to ask here. This is ridiculous.
Pathetic.
Wow, I didn’t knew there was such thing as a fake papers generator.
“Internally, the tool bases on many techniques of the Natural Language Processing research field, including sentiment analysis, part-of-speech tagging, and named entity recognition.”
This is insane how far technology is evolved that it is already able to detect so much stuff. I think everything evolves in this way, even writing articles in the future will be with automated tools in my opinion.
thanks for this article
There are algorithms for detecting fake papers and they work fairly well, just like there are algorithms for detecting plagiarism. The publishers seem to not use them.
Lee Giles