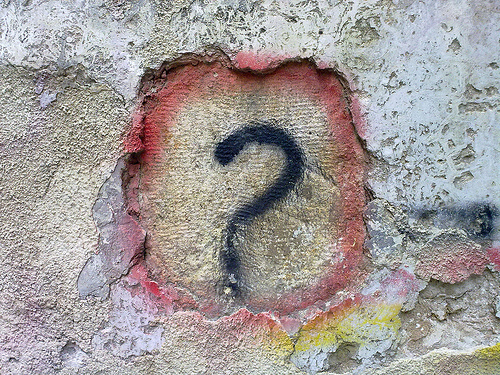
Another installment of Ask Retraction Watch:
Recently I heard a graduate student was told by their advisor, ‘Don’t do a t-test, it’s not publishable.’ This seems ridiculous to me as the t-test is a robust test to aid in answering a hypothesis. So my question is: is a t-test no longer publishable? And if so, is this true for higher tiered journals, or all peer-reviewed journals?
I would very much appreciate hearing the opinions of your readers on this issue – do they feel they need to run more ‘elaborate’ statistics (e.g., multivariate, modeling, etc.) in order for their research to be publishable? And if so, do researchers knowingly violate the assumptions of these more elaborate statistical tests so they can be ‘publishable’?
Please take our poll, and comment below.
I would genuinely like to hear the advisor’s reason for making that statement, and others’ take on this. I’m no stats whiz, but from what I’ve been taught, there are no “better” or “worse” (read: more publishable or less) statistical tests, only those that are applied “correctly” or “wrongly” (e.g. with regard to underlying assumptions) to a data set, given the hypothesis. If a t-test is appropriate, I personally don’t see why it shouldn’t be publishable.
I have a feeling the advisor’s reason is that a t-test is way too simple, and that you need to use a more complicating model for an analysis to be interesting and useful. However, this will all depend on the research question. If the question can be answered by just comparing two means, adding unnecessary variables in the model simply complicates the interpretation and veers you away from the main purpose of the study.
Silly question. The simplest and most appropriate statistic should always be used. Statistics like all other aspects of science can be abused or used incorrectly. Reviewers of a paper should decide if the appropriate statistic was used correctly. It is next up to the journal to review statistics.
Yes, silly question. Without knowing more about the original circumstances, we don’t know why the adviser said what they did. He could have meant “don’t do a t test because these results aren’t publishable no matter what the t test says”, rather than “don’t do a t test because t tests aren’t publishable”.
Some possibilities:
1) There’s something else wrong with this experiment, so don’t waste your time analysing it further. (e.g. if the student buggered up the control samples, there’s no use testing for a difference between treatments A and B)
2) I’ve just realised that this observation is a simple consequence of $previously-known-result, so it’s not publishable even if it’s significant.
3) You don’t need to do a t test to see that the result isn’t significant (e.g. if the supervisor is better at mental arithmetic / estimation than the student)
4) There’s a known confounding variable here, so a simple t-test is inappropriate
It would help to specify, in which context the t-test (or a correlation, which is the same) was used. If it was used in an true experimental design for testing the difference between two experimental groups, then it is appropriate, of course. However, if it was used in a correlational design in one of the behavioral sciences, e.g., for testing the difference between men and women in their reported well-being, then the reviewer was correct in my opinion. Such a simplistic model has 99.99% chances to be severely misspecified, and hence wrong. I love a quote from Paul Meehl that addresses this issue: “In social science, everything is somewhat correlated with everything (“crud factor”), so whether H0 is refuted depends solely on statistical power. In psychology, the directional counternull of interest, H*, is not equivalent to the substantive theory T, there being many plausible alternative explanations of a mere directional trend” (Meehl, 1990, p. 108)”.
Ilan expressed well my own thoughts about the t-test. In the behavioral and social sciences it is more difficult to find applications for the use of t-tests today than it was 50 years ago.
I’d go further to say that it’s often difficult to create an experimental design today that addresses some of the most difficult and engaging questions that are out there. Less hard sciences have built up a core of understanding that better acknowledges the complexity of behavior, context and history and which makes it more difficult to craft designs that use a simple t-test, IMO.
The question is horrifying — the t test is actually the best of all statistical tests. It is simple, easily understood, and very robust with respect to the conditions necessary to apply it. As a reviewer I might actually consider rejecting a paper (well, asking for revision) if it failed to use a t test in conditions where it would be possible.
Actually I generally reach for Mann-Whitney U or Wilcoxon signed-rank when I’m doing the kind of thing that one usually uses a t-test for.
If your data are normally distributed, you’re doing it wrong.
Never use a non-parametric stat when a parametric one will do.
It doesn’t even need to be normally distributed. All you need is bounded variance and a sufficient amount of data.
But to correct the assertion slightly, it isn’t that a non-parametric stat should never be used, it’s that it tests a different (and usually less important) aspect of the data. Essentially those nonparametric tests compare the median, whereas a t test compares the mean. The nonparametric tests also tend to be weaker in many cases.
I almost never do the non-parametric tests. While the simple test can be used, there are no approaches for non-parametric tests in a regression sense – examining a difference while controlling for other variables. The simple difference is only the beginning usually, yet the non-parametric approaches ONLY allow for the unadjusted test, which is often of interest, but of bounded interest.
Yes, there are. An exceedingly widely used non-parametric regression is e.g. Cox proportional hazards regssion.
My guess is that it was not publishable because it was not the appropriate test. The most common reasons why t-tests are not appropriate are multiple comparisons or circumstances where the data are likely not to be normally distributed.
In psychology, I’ve heard some grumbling this effect. You’re unlikely to get a paper where the only inferential statistic is a single t-test published in a decent journal (unless they do brief reports), but they might well accept a three-study paper where each one just does a t-test. On the other hand, a paper that reports a single structural equation model, factor analysis, multiple regression, etc., might only need a single study to be published by the same journal.
So I don’t know if this is a shorthand approximation of “your paper needs to have more information than would usually be encapsulated in a single t-test in order to be published by a good journal,” or if editors and reviewers literally reject papers because they use t-tests regardless of the information those tests convey. That said, Psychological Science now explicitly prefers authors to report CIs and deemphasise t-tests, but I don’t think that’s what’s being referred to here.
The t-test is useful and very robust in many circumstances. Like any statistic, it is just a tool that has to be used properly
We would need more information. If the t-test shows something worthwhile, then it is obviously publishable in any journal.
But in my work and experience (biology, biochemistry, chemistry) formal statistical tests are very rarely done. The reason is that your results should be so obvious that a statistical test is not necessary. For example, if you are developing a catalyst, and you need a statistical test in order to prove that your results differ from background, then you don’t really have a worthwhile result.
What about the case of having a catalyst which is supposedly just like another catalyst? How do you establish the correspondence? I occasionally attend biology talks, and these have many pictures. The pictures are shown, and the person says “As you can clearly see that X is darker than Y” Well, I can’t clearly see it. This is one reason why biology is in so much trouble, and why reproducible results are often hard to get. It’s so subjective. I never see a quantitative amount on these blots. I never see “we did the blot 12 times, and of the 12, 11 of the 12 showed the relationship”. N=1, subjective examination, lack of quantitative rigor, and you have a whole bunch of crap in many cases.
That’s a very interesting statement, at least for me as a behavioral scientist who depends on statistical tests to see the signal in all the noise. Apparently, dependence on statistical inference is not equally distributed across the sciences. It’s probably less important in all those cases where you can directly observe the actual process the gives rise to a result (e.g., chemical reactions). This is something that you only very rarely see in the behavioral sciences where the outcomes that we measure are far downstream from any single causative process and rather represent the joint mass effect of a myriad individual processes (e.g., neurons in interaction).
In any event, I clearly envy you for being able to see an effect directly, without having to resort to statistical inference, be it Bayesian or frequentist.
There are plenty of situations in which a t-test is not appropriate. In particular, many persons in basic biology use repeated t-tests to examine the various levels of a multi-factor design. This is not appropriate, but is a common issue. However, when there are two groups, the variable of comparison is gaussian, and the modest assumptions for the test are met, the t-test is a perfectly acceptable result. I do the t-test or the equivalent in many cases. One point, however, is that many situations in which a t-test might have been considered 20 years ago are no longer appropriate. If the data are Poisson, binomial, or taken from a larger design, the t-test is not appropriate. As others have stated correctly, the issue is not the test per se, but that the test has been chosen correctly.
as is suggested by the behavior of this advisor, i think that there’s a bias towards the use of nonparametric tests, not so much because they are better as because they have a lower burden of proof for publishing a paper. if you have 3 reviewers, you can depend on one of them getting fussy about normality. however, in a properly controlled experiment with even a modest sample size (which we can do in biology, my discipline), there is no better test than the welch t-test for the null hypothesis that the means of two samples are the same. the most commonly substituted nonparametric tests (i.e. wilcoxon, u-test) test a vague, possibly meaningless null hypothesis of whether distributions are different.
to directly answer this person’s questions, i say that if you need elaborate statistics to make an argument, your argument is probably wrong. we do of course need to control for as many exogenous variables as possible, but these controls are in no way inconsistent with simple statistical tools.
granted, it would be nice if we could dispense with hypothesis tests and p-values altogether, and focus instead on things that actually matter in real life, like effect sizes and informational content of variables. but as long as we live in frequentist world, we might as well use the tool that is best.
I am hoping (?) that the advisor had a specific reason for making that comment- as in, it was an inappropriate statistical test to use for the data or for the research question. I have seen many recently published papers, and published many of my own, using t-tests. Now, I do know that certain fields tend to lean towards t-tests more than others, but this is certainly no reason not to use a t-test if it is the most appropriate test for the data and RQ. If you have good data, meaningful and accurate results, and your findings can contribute to your field…publish it!
And what is the link to retractions? Are journals now going to start retroactively retracting papers based on if they used the t-test, or not? If so, I would say that half of the literature in my field would be retracted immediately.
The dudes/dudettes supervisor is incorrect. The t-test would only be incorrect if there were more than two groups being compared and they were doing repeated T-tests instead of an ANOVA
That is exactly right. I’ve read many papers where there are more than two samples (one control/several treatments), and comparisons are made by running multiple t-tests between each treatment and the control, instead of an ANOVA test. My first lesson in Statistics 101 was that you can’t do that.
There’s nothing wrong using multiple t-tests when the p-values are corrected using one of the many available methods (see e.g. http://stat.ethz.ch/R-manual/R-patched/library/stats/html/p.adjust.html for an overview), but your p-values will explode if you have many groups and an ANOVA may be more appropriate (*if* its requirements are met).
If you are responding to the exact situation of Cardinal, I disagree that correction is all that is needed. When multiple t-tests are used when a designed set of contrasts are used, the correction of p-values for multiple testing is not the problem. The problem is specifically the use of the t-test which uses a different and varied standard error for each test. It is easy to construct a demonstration in which several groups in a large experiment have exactly the same numeric difference, but which show a difference in significance due to the error in each group. If the t-test is used, inconsistent and incompatible results will occur.
Could you please construct such a counterexample, and post it here?
In some situations it is also helpful to report the effect size, which can easily be estimated from the samples to get a more intuitive measure of the differences in the underlying distribution, see for example Wolfe & Hogg, “On Constructing Statistics and Reporting Data,” The American Statistician, 25(4):27-30, 1971.
In my field (astronomy) If I see a T-test (or any of the other frequentist tests mentioned in these comments, parametric or non-parametric), I immediately raise an eyebrow. It’s not that those tests are problematic per se, or that Bayesian methods are better (although I think they usually are). It’s rather that, if an astronomer could be so ignorant of community norms to be using a T-test in 2013, I question how sophisticated a statistical thinker they are. That means I either need to scrutinise the paper more closely, or skip it altogether and move on to the next paper in my reading pile (usually the latter.)
Just curious, what are the community norms in astronomy in 2013?
Bayesian statistics.
I agree with:
Dan Zabetakis: “…your results should be so obvious that a statistical test is not necessary. For example, if you are developing a catalyst, and you need a statistical test in order to prove that your results differ from background, then you don’t really have a worthwhile result.”
max: “…if you need elaborate statistics to make an argument, your argument is probably wrong.”
And let’s add to this that, practically, “everything is somewhat correlated with everything”, which, in other words, means that if you start increasing the size of the sample, you will end up with finding the difference with control. (In other cases, statistics is good at not finding difference with your theory/hypothesis.) The real question is always boils down to: What difference does your difference make? There is no statistics that excludes human element at this crucial point.
Instead of teaching statistical methods, universities should teach common sense and keen perception. For instance: give the students numbers and ask to convert them into graphics (we are much better in understanding graphic images than numbers), and ask them to make conclusions. At the same time, have statistical calculations to show whether their perception is close to calculation. I never remembered any statistical methods, never needed or used them and, if you say – being ignorant, developed a hostility to statistics. But, I think that I am fully able to make correct estimates from the graphics and never to make stupid conclusions.
The books on “How to lie with statistics” always start with graphs. It is MUCH easier to lie with graphs because there are no actual numbers there which can be checked. If we have scientific fraud today, and we do, it is HIGHLY concentrated in the areas of science which use non-statistical methods like biology. Copying figures, faking figures – case after case, no statistics but lots of pictures. I guess common sense involves using pictures, and when you don’t have them, just making them up.
I kind of think that you are seeing lots of fraud in biology and probably in medical science but attribute it incorrectly to the lack of numbers.
You say: “It is MUCH easier to lie with graphs because there are no actual numbers there which can be checked.” Here you postulate the presence of lie, and then blame the graphs. You should blame liars. Also, numbers are not erased in good graphs.
Then, you say that fraud “is HIGHLY concentrated in the areas of science which use non-statistical methods like biology.” Yes, fraud is highly concentrated in biology and medicine, but especially in the papers that are using statistical methods. It’s these methods that allow to cover up fraud. Example: the flu vaccine was “proved” effective in papers, showing the effect to be statistically … Years latter, it was admitted that the effect itself was miniscule: 6 days of flu instead of 7 days without vaccine, and the vaccine does not prevent infection.
Then you say: “Copying figures, faking figures – case after case, no statistics but lots of pictures. I guess common sense involves using pictures, and when you don’t have them, just making them up.” Again, you are against using pictures because crooks are forging pictures. They forge numbers as well. There is a difference though: it’s much easier to detect forgery in pictures. It’s almost impossible in numbers.
Finally: you can describe a facial expression on the photograph. Try to do it from a picture digitised on a grid.
One of the reasons, and the chief reason, why so many biology papers are now fraud, is in the delusion, introduced by physicists in early ’60s, that biological processes are stochastic in nature. Every biological mechanism since that time was “explained” by a “Stochastic Model of …………..”; thousands of such garbage were published. A statistician became an indispensable co-author, well, I don’t go into the details. In short, biological laws became something nobody wants even to look for.
Statistical significance is not the same as practical or clinical significance. This is widely known. Statistical significance is but one type of criterion for importance or clarity of effect. In particular, with situations in which numbers are large, the utility of the notion of “statistical significance” is sometimes questionable. But that is the problem with absolutist positions.
As to the detection of fraud in numbers, it’s really a matter of evaluation. Yes, a clever person can fake numbers well. Mostly those who fake are not clever, and the fakery is pretty obvious. Patterns are produced, and these are not what is seen in real data.
As to the ability to detect fraud in images, if it is so easy, why are there so many retractions involving images? Why did they get published in the first place?
You should never use a t-test. Actually you should never use hypothesis tests at all. Just report Bayes-factors!
blech…the subjectivity of it all.
Bayes-factors are not subjective, priors are. But if you come up with an “objective” interpretation of a p-value, all the better…
The value of the Bayes factor depends on the choice of prior, right? And even putting that aside, interpretation of a p-value would seem to be at least as objective as that of a Bayes factor.
Well, if you compare two specific hypotheses, the Bayes factor depends only on the data. And yes, the p-value has an objective interpretation, it just doesn’t tell you anything about what you are really interested in. In practice, p-values are used to “measure” the evidence against the null, which is (a) a subjective procedure and (b) a flawed one.
As I understand it, “hypothesis” here means not just a model with parameters but also a distribution for those parameters, which is not generally “objective” in any sense. Then there are those non-objective-sounding scales that tell us that a Bayes factor of 1-3 is “barely worth mentioning”, a Bayes factor of 3-10 is “strong”, and so on, but I don’t know whether and how they are used.
What I had in mind was a comparison of H0 and some specific H1. If H1 is not specified, then you need a prior distribution for its parameters, right. But your prior hypothesis odds do not enter the Bayes factor. Of course you cannot get rid of all “subjectivity”, neither with Bayes nor with p-values. After all, a person’s prior beliefs depend on her prior information and even in objective Bayesianism there is no such thing as “objective prior information”. I just think that if you *have* to be subjective in this sense, then you should better choose the scientifically sound way among the available ones.
In that case the Bayes factor is just the usual likelihood ratio, right?
I suppose this isn’t the place for an extended discussion of such things, so I’ll leave it at that.
Agreed! (both)
In my humble opinion, the t-test is a left-over from the days in which male, white scientists tried to press their capitalist stamp on science. To hell with it! What we urgently need is a new, progressive, liberating brand of statistics that can be used to support any kind of progressive hypothesis.
(With a nod to Alan Sokal.)
As much as I like your comment, can we please keep the science war stuff out of here. We have a common and serious problem after all, i.e. misconduct, no matter whether scientific or scholarly.
You are welcome to see the qualitative stuff as pure non-sense, but I’d rather see more discussion on how serious issues like data fabrication could be evaluated in the humanities and related fields. For instance, since we are not dealing with “big data”, could it be possible to mandate the publication of all interview samples etc.?
We have such a liberating brand of statistics. Bayesian statistics recognizes that *all* priors are social constructs. There is no reason that the likelihood framework, with its hidden implicit uniform improper prior, should be accorded a privileged position. With proper choices of priors, we can find support for any of those hypotheses.
(With apologies to foobar)
“Statistical significance is not the same as practical or clinical significance.” Yes, and that was my point. But my point is also that often the work is taken as “progress” on the basis of statistical significance, while it is in fact just a garbage. The statistical significance here is distracting attention and helping fraud.
“In particular, with situations in which numbers are large, the utility of the notion of “statistical significance” is sometimes questionable.” You mean size of the sample? I don’t understand this. If p value is the same, why large numbers are worse? Or you mean that with high dispersion you need large sample and data for individual measurements cannot be trusted? Don’t understand anyway, need better training.
“As to the ability to detect fraud ..etc.” Statistics is valuable, first of all, to the author himself. It was not invented to fight fraud.
… of course you can’t publish a t-test… Student already did! (hahaha) but the real question is why he isn’t cited in every paper that uses his test…
I think the right question would be whether the paper is publishable if t-test is the ONLY statistics used in the manuscript.
I think the greater threat of fraud is not so much in the choice of test, because, like economics, there are different schools of thought as to which is a more appropriate or robust test. What is of more concern is when a scientist claims to have conducted statistical analyses, and then reports none, or reports contradictory assessments with apparently the same test. These papers need to be called out because they likely represent fraud and/or data manipulation. In fact, when such black-on-white contradictions actaully exist, it also reflects the slack nature of the “peer” review.