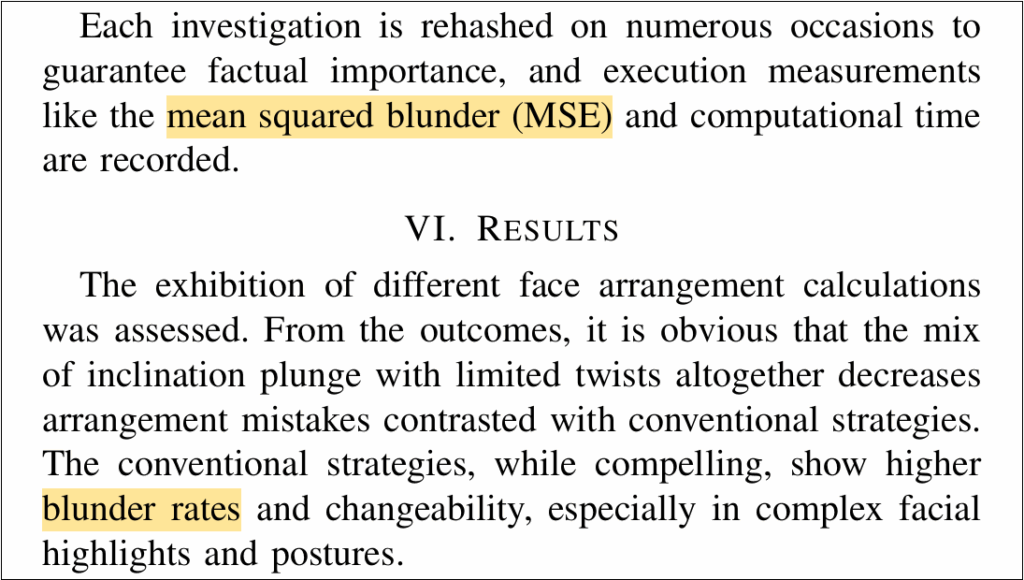
An expert in AI at Google has admitted he used the technology to help write a preprint manuscript that commenters on PubPeer found to contain a slew of AI-generated phrases like “squared blunder” and “info picture.”
The paper, “Leveraging GANs For Active Appearance Models Optimized Model Fitting,” appeared on arXiv.org in January but was withdrawn April 7. The author, Anurag Awasthi, is an engineering lead in AI infrastructure at Google. In a PubPeer comment, he described the paper as a “personal learning exercise.”
In March 2025, sleuth Guillaume Cabanac, creator of the Problematic Paper Screener, pointed out in a PubPeer comment the paper included several tortured phrases. These phrases indicate AI use and occur when large language models try to find synonyms for common phrases. In Awasthi’s paper, “linear regression” became “straight relapse,” and “error rate” became “blunder rate,” among others.
Awasthi replied to the comment saying “phrasing issues were unintentional artifacts from an earlier revision where automated tools were used to rephrase for variety.”
Another PubPeer comment pointed out similarities between Awasthi’s preprint and a 2016 paper by different authors. Aside from containing similar structure, Awasthi’s paper uses much of the same language.
Awasthi again replied on PubPeer reiterating the overlap was an “unintended artifact.” His comment reads, in part:
This preprint was intended to explore a new idea—to the best of my knowledge—and I shared it early to test its relevance. The project initially began as a personal learning exercise but sensing a possible novelty, turned into a publication attempt. The layout and literature review, drafted with the help of AI-assisted tools, ended up too close in phrasing to earlier work—particularly the one you’ve highlighted.
After receiving further criticism about the undisclosed AI use, Awasthi replied that he “clearly underestimated the seriousness of preprints.”
He responded to our request for comment by directing us to the Google press office, which did not respond.
Like Retraction Watch? You can make a tax-deductible contribution to support our work, follow us on X or Bluesky, like us on Facebook, follow us on LinkedIn, add us to your RSS reader, or subscribe to our daily digest. If you find a retraction that’s not in our database, you can let us know here. For comments or feedback, email us at [email protected].
Ironic
It’s interesting. A lot of schools – certainly at the primary and secondary level – teach you not to repeat phrases, to mix synonyms up so as not to bore the reader. But technical writing (legal, scientific) is the opposite – you pick a particular word/phrase with a precise meaning, and you KEEP USING that word/phrase whenever you need it, because if you use something different, the reader will be misled to believe you intend something significantly (meaning, notably, definitionally) different.
Ref: https://en.wikipedia.org/wiki/Elegant_variation
The replies from the author were constructive. Rather than focusing on particular cases, we should really try to reflect what happens in this AI age if we allow the scientific literature base to be poisoned with nonsense, starting from terminology. You already see the effects; Googling “herbal language processing” gives you a confident but wrong answer by Google’s LLM. The same is going on elsewhere; the other day there was a good piece in the Register about LLMs hallucinating software package names that are actually malware, and here too it is driven by data poisoning.
Ummm, the author’s explanations make no sense; just attempts to misdirect from the core problem.
The author didn’t write the paper and add errors, AI generated it.
Word blunders and hallucinations are part of the core functionality of LLMs.
Please authors, try using actual human intelligence and not some knock-off pseudo intelligence that you can buy on-line.
He admitted his foul mistake and withdrew his pre-print, which in my books is more than adequate. Otherwise I agree with you very much. I’d like to see a statement from Google too because misinforming (“hallucinating”) about science can have dire consequences for the world and people who cannot evaluate outputs (cf. foremost medicine and healthcare but many more too). Their seatch engines were never perfect but now it is really dangerous.
If you think the so-called author’s response in this case was “more than adequate,” I wonder what you would consider “adequate.”
Don’t hold your breath for Google to achieve AGI anytime soon. Just regular human intelligence would be an improvement.
No way serious researcher would make errors like those pointed out: “linear regression” became “straight relapse,” and “error rate” became “blunder rate,” among others.
He doesn’t claim to be a serious researcher, he claims to be an AI expert.
True story my editor suggested rephrasing which did involve replacing terms… Sadly, it generates more work instead of saving us time, since I had to read and reply to all these “suggestions”.
Can’t believe it, it must be a joke. Any modern LLM just can’t generate such text, it’s simply impossible. It will tell you right away that “straight relapse” isn’t a synonym for “linear regression”. It “knows” it’s incorrect, that it makes no sense at all. Perhaps the author was testing some sort of simpler, smaller LLM.
P.S. Just for fun, I asked an LLM rewrite my comment in a professional manner, and you can see how perfectly it did it:
It appears highly improbable that the text equating “straight relapse” with “linear regression” originated from a contemporary Large Language Model (LLM). Current LLMs generally possess a robust understanding of established statistical terminology and would typically recognise “straight relapse” as nonsensical in this context, correctly identifying “linear regression” instead. Such a fundamental error is inconsistent with the expected capabilities of modern architectures. So, a more plausible explanation might involve the utilization of a less sophisticated or perhaps smaller-scale language model for the generation of this output.
Excellent insight! It was insightful to ask for LLM input. Your comment gave me a new perspective (i.e., was very helpful!)
In Total agreement with you. It’s a bad joke. Come on, everyone uses LLM in writing. No one can control PHD students, who previously publish nonsense. IMHO, 90% of papers are nonsense. And now we’re blaming LLM.
I feel like calling it “AI” is being overly generous. This is not the result of an LLM, but of some sort of simple synonym-replacement script.
The exact same phrases as seen here have been appearing in (way too many) papers for over a decade now (the oldest example I’ve seen was from 2013). The most likely suspect is Spinbot or one of its clones.
Highly relevant reference:
Cabanac et al. (2021), “Tortured phrases: A dubious writing style emerging in science. Evidence of critical issues affecting established journals”, arXiv preprint,10.48550/arXiv.2107.06751
So it appears that AI professionals are so trusting of their LLM outputs that there is no need to proofread the textual output. And we wonder why AI hallucinates and makes really fundamental ‘blunders’. If the output is not being checked adequately by the very ppl who should be doing the quality control why are we surprised that there are these glaring errors in textual output?