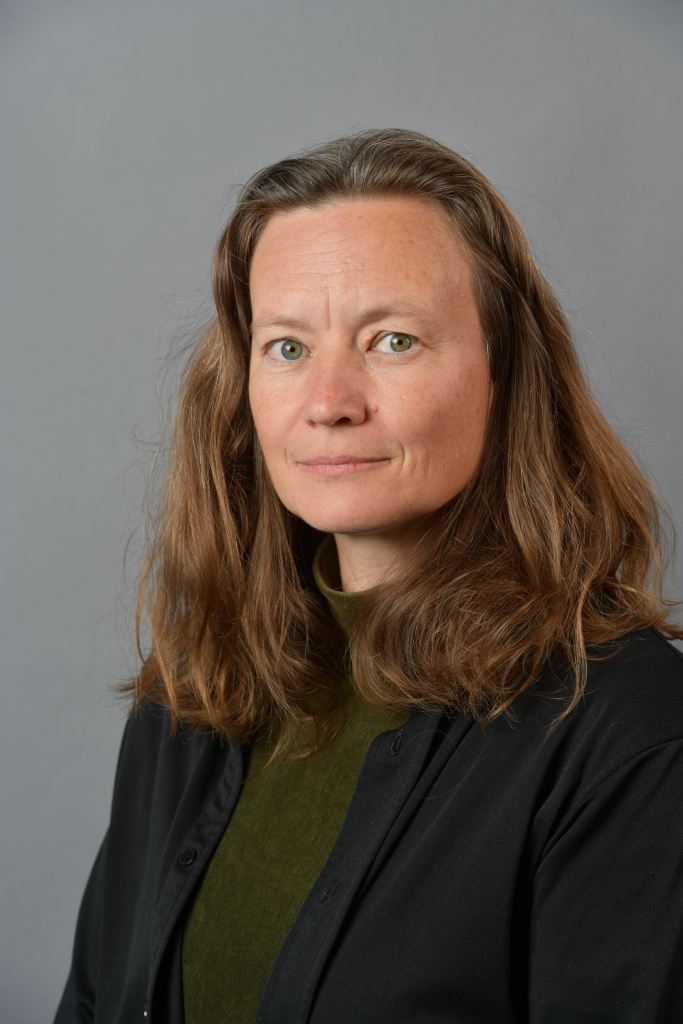
Some Retraction Watch readers may recall that back in 2012, we called, in The Scientist, for the creation of a Transparency Index. Over the years, we’ve had occasional interest from others in that concept, and some good critiques, but we noted at the time that we did not have the bandwidth to create it ourselves. We hoped that it would plant seeds for others, whether directly or indirectly.
With that in mind, we present a guest post from Anita Bandrowski, who among other things leads an initiative designed to help researchers identify their reagents correctly and has written for Retraction Watch before. She and colleagues have just posted a preprint titled “Rigor and Transparency Index, a new metric of quality for assessing biological and medical science methods” in which they describe “an automated tool developed to review the methods sections of manuscripts for the presence of criteria associated with the NIH and other reporting guidelines.”
Science seems to publish many things that may be true or interesting — but perhaps not both. Ideally all of science should be both true and interesting, and if we were to choose one, my hope would be to choose true over interesting.
We have had a way to measure interesting for many years, the Journal Impact Factor. This controversial yet frequently used metric for the things that really matter to scientists such as whether they will get a job or not, has ruled academia for decades. Of the many problems that have been pointed out with the metric, the most serious in our opinion is that it measures popularity. It may well be that popularity is associated with quality, but from what we have seen on Twitter or Facebook, popularity is likely to have little to do with quality.
Unlike the software industry, which has a method to benchmark development assessing quality of companies based on reasonably impartial metrics (see DORA), scientific papers thus far lack systematic measurement.
The National Institutes of Health and many top journals, looking at objective evidence, have settled on a number of aspects of studies that are the hallmarks of quality, though in no way do they guarantee reproducibility. These are largely found in the experimental methods, the section of the paper reduced to obscurity and facing extinction in many of the most “interesting” journals, such as Science. These aspects include things like:
- Did the authors account for investigator bias in the study?
- Did the authors discuss the metrics used to select group size?
- Did authors address how subjects were put into groups?
- If someone tries to find the resources and reagents used in the study, did the authors leave sufficient information for them to do so?
These are all obviously not going to be applicable to all studies equally nor to all journals equally, but in general these sorts of criteria are represented in various checklists and guidelines.
The answer to these questions, however, is difficult to gauge. Scoring the answers would be equally difficult, and determining if the answers are appropriate for the study would take careful reading of the paper by an expert, i.e., peer review. Of course we know from various back room conversations with editors that they have a really hard time getting reviewers to even look at the methods.
But we are currently in the era of AI. Perhaps this technology can help? AI –or a group of technologies including classifiers and text mining — has indeed come a long way recently. Still, it is far from “taking over the world,” or more importantly for our case, understanding what we mean when we publish scientific papers. That means some of the questions above, including are the authors addressing investigator blinding adequately in the context of the paper, are still pretty far out of reach of this technology. However, the technology can tell us with a measured certainty whether authors are addressing investigator bias.
Introducing SciScore
We built a tool called SciScore, based on various classifiers and neural networks, which can detect whether a given sentence matches the prototypical statement about investigator bias. The tool is also aware of various catalogs of millions of reagents and can compare the reagent description with all of those and tell us whether or not it matches a known reagent at a high level of confidence. None of these matches are perfect, but the tool does appear to do a job that human reviewers do not seem to want to do with any consistency, to say the least, and it is completely unbiased for any given paper (please note, not all criteria will be applicable to each paper, but the tool is not aware of the applicability of the criteria only the presence or absence of matching text).
The tool tallies the things it finds (is a sentence about investigator blinding found in the paper?) and compares this number to the things it expects to find (expectation: there will be a sentence about blinding) and gives a score between 1-10, based roughly on the proportion of expected and found.
This score for a paper is certainly flawed, there are rates of false positives and false negative results, so any one item may be incorrect at a known frequency. It is probably not fair to expect that all criteria are met for every study. Here we will plead ignorance based on the state of the technology as the question whether a study should address certain criteria is currently too difficult to answer using the tools at hand, but perhaps some smart computer scientists can answer that question at some point.
However flawed, what we do have is a brand new metric that covers about 30 aspects of quality of a study and gives a simple number.
So we were bored, and decided to grade the entire accessible biomedical literature with this tool and wrote up the results and just released the preprint to BioRxiv. So what are the outcomes? Well, for those members of Retraction Watch that are paying attention, they are going to be relatively unsurprising. The literature can certainly be in somewhat better shape.
In 1997, scoring 1024 papers, 10% of studies addressed how group selection occurs (randomization metric), that same year the power calculation, which is a simple statistical formula to determine how large groups should be was detected in 2% of papers. Letting the reader know which sex animals are was reported in 22% of papers, and antibodies were findable about 12% of the time. This is not a great result.
We at the RRID initiative have been passionate about fixing some aspects of this problem, especially for antibodies! We can and do, boast that RRIDs are present in over 1,000 journals and that we have several hundred thousand RRIDs that have been put into papers by diligent authors. Authors have been amazingly helpful in tagging their reagents when asked to do so by journal editors, and sometimes out of the goodness of their hearts because they want to at least get the “ingredient list” for a given paper nailed down. But has the RRID literature effected the overall quality of reporting of the antibodies?
Have scientists read the various guidelines and changed the way they report? The good news: Some have. Of the metrics above, the 142,841 2019 papers scored are a bit better than their 1997 counterparts: randomization has gone up from 10-30%, power calculation from 2-10%, reporting sex from 22-37%, and antibodies are findable 43% of the time compared to 12% in 1997. So, on the one hand we can congratulate ourselves for doing better, but on the other hand half of papers don’t tell you the sex of experimental subjects and how they are divided into groups. Unfortunately this is not surprising, because smaller studies with more targeted samples of papers have shown basically the same thing. We are not doing well in terms of addressing criteria for rigor, much less addressing these in a manner that is appropriate.
So how does that new number compare to the other simple number, the journal impact factor, which essentially takes into account the number of citations that a paper gets? It turns out that there is no correlation, so some high impact journals tend to do really well, including many of the Nature Research journals, because it seems that they are able to enforce their checklists. For example, Nature Neuroscience’s score went from 3.58 in 2008 to 6.04 in 2019. Some high impact journals such as PNAS, which periodically make various decrees about rigor and reproducibility, do not appear to follow any of the recommendations as evidenced by the lack of change of their composite score, which continues to hover around the low 3 range. Clinically focused journals tend to do better overall, most likely because their checklists have been ingrained in the reporting of clinical trials for decades. Chemistry journals, tend to score very poorly, but one might argue that these should not be compared to the biomedical literature because the techniques are so different.
The take-home message? We can all probably do better and checklists, if followed, can help.
Like Retraction Watch? You can make a tax-deductible contribution to support our work, follow us on Twitter, like us on Facebook, add us to your RSS reader, or subscribe to our daily digest. If you find a retraction that’s not in our database, you can let us know here. For comments or feedback, email us at [email protected].
The problem of “experimental methods, the section of the paper reduced to obscurity and facing extinction” is real, and under-recognised. It really should not be a problem at all, if the journal allocates generous space for supplementary material (as all should). One rather perverse problem is that when authors publish a series of papers with similar (but gradually evolving) methodology, a full description of the methodology might be detected as self-plagiarism by anti-plagiarism tools.
We have struggled to convey to journals that methods should be completely plagiarized! Some still don’t buy the argument, but many do.
There are tools like protocols.io that make the process of reporting a full protocol much easier. I, for one, hope that those efforts grow.
Methods should be completely plagiarized? You mean to say that authors should be allowed to copy-paste _portions_ of Methods sections from one paper to another when such textual material describes identical processes, equipment, etc., correct?
I may be wrong, but it seems to me that few methods are truly identical in their entirety from one experiment to the next even when the subsequent experiments are planned as exact replications of earlier ones. I would go as far as suggesting (as I believe others have) that, in fact, it is the failure to describe those nuanced differences in ‘identical’ methods that attempt to replicate others’ work that may, in part, account for the reproducibility crisis in some sectors of science.
Yes, indeed I am taking a bit of a poetic license. However, in the case that a person is attempting to reproduce a study, ideally the methods should be identical or nearly identical.
I think that the goal is to not have to worry about plagiarism in the methods section because it is a waste of time to think about how many different ways you can say “I transferred the tissue to buffer for 3 minutes”. Nor, is that a productive exercise.
The nice people who created protocols.io knew this and have a way to “plagiarize someone’s protocol”, it is called forking (like in GitHub). So I can today use the method of someone, I can change step 3 from 2 minutes in buffer to 3 minutes in buffer and the protocol is exactly what I used in my paper. I can get a DOI for that and I can use it in my manuscript. The document is linked to the previous document and so I give credit to the creator.
In all, I think that this is the right way to think about methods.