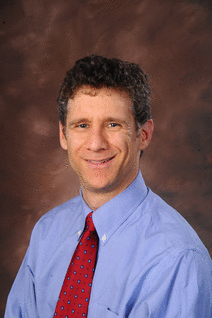
Doing research is hard. Getting statistically significant results is hard. Making sure the results you obtain reflect reality is even harder. In this week’s Science, Eric Loken at the University of Connecticut and Andrew Gelman at Columbia University debunk some common myths about the use of statistics in research — and argue that, in many cases, the use of traditional statistics does more harm than good in human sciences research.
Retraction Watch: Your article focuses on the “noise” that’s present in research studies. What is “noise” and how is it created during an experiment?
Andrew Gelman: Noise is random error that interferes with our ability to observe a clear signal. It can have many forms, including sampling variability by using small samples, or unexplained error from unmeasured factors, or measurement error from poor instruments for the things you do want to measure. In everyday life we take measurement for granted – a pound of onions is a pound of onions. But in science, and maybe especially social science, we observe phenomena that vary from person to person, that are affected by multiple factors, and that aren’t transparent to measure (things like attitudes, dispositions, abilities). So our observations are much more variable.
Noise is all the variation that you don’t happen to be currently interested in. In psychology experiments, noise typically includes measurement error (for example, ask the same person the same question on two different days, and you can get two different answers, something that’s been well known in social science for many decades) and also variation among people.
RW: In your article, you “caution against the fallacy of assuming that that which does not kill statistical significance makes it stronger.” What do you mean by that?
AG: We blogged about the “What does not kill my statistical significance makes it stronger” fallacy here. As anyone who’s designed a study and gathered data can tell you, getting statistical significance is difficult. And we also know that noisy data and small sample sizes make statistical significance even harder to attain. So if you do get statistical significance under such inauspicious conditions, it’s tempting to think of this as even stronger evidence that you’ve found something real. This reasoning is erroneous, however. Statistically speaking, a statistical significant result obtained under highly noisy conditions is more likely to be an overestimate and can even be in the wrong direction. In short: a finding from a low-noise study can be informative, while the finding at the same significance level from a high-noise study is likely to be little more than . . . noise.
RW: Which fields of research are most affected by this assumption, and the influence of noise?
AG: The human sciences feature lots of variation among people, and difficulty of accurate measurements. So psychology, education, and also much of political science, economics, and sociology can have big issues with variation and measurement error. Not always — social science also deals in aggregates — but when you get to individual data, it’s easy for researchers to be fooled by noise — especially when they’re coming to their data with a research agenda, with the goal of wanting to find something statistically significant that can get published too.
We’re not experts in medical research but, from what we’ve heard, noise is a problem there too. The workings of the human body might not differ so much from person to person, but when effects are small and measurement is variability, researchers have to be careful. Any example where the outcome is binary — life or death, or recovery from disease or not — will be tough, because yes/no data are inherently variable when there’s no in-between state to measure.
A recent example from the news was the PACE study of treatments for chronic fatigue syndrome: there’s been lots of controversy about outcome measurements, statistical significance, and specific choices made in data processing and data analysis — but at the fundamental level this is a difficult problem because measures of success are noisy and are connected only weakly to the treatments and to researchers’ understanding of the disease or condition.
RW: How do your arguments fit into discussions of replications — ie, the ongoing struggle to address why it’s so difficult to replicate previous findings?
AG: When a result comes from little more than noise mining, it’s not likely to show up in a preregistered replication. I support the idea of replication if for no other reason than the potential for replication can keep researchers honest. Consider the strategy employed by some researchers of twisting their data this way and that in order to find a “p less than .05” result which, when draped in a catchy theory, can get published in a top journal and then get publicized on NPR, Gladwell, Ted talks, etc. The threat of replication changes the cost-benefit on this research strategy. The short- and medium-term benefits (publication, publicity, jobs for students) are still there, but there’s now the medium-term risk that someone will try to replicate and fail. And the more publicity your study gets, the more likely someone will notice and try that replication. That’s what happened with “power pose.” And, long-term, enough failed replications and not too many people outside the National Academy of Sciences and your publisher’s publicity department are going to think what you’re doing is even science.
That said, in many cases we are loath to recommend pre-registered replication. This is for two reasons: First, some studies look like pure noise. What’s the point of replicating a study that is, for statistical reasons, dead on arrival? Better to just move on. Second, suppose someone is studying something for which there is an underlying effect, but his or her measurements are so noisy, or the underlying phenomenon is so variable, that it is essentially undetectable given the existing research design. In that case, we think the appropriate solution is not to run the replication, which is unlikely to produce anything interesting (even if the replication is a “success” in having a statistically significant result, that result itself is likely to be a non-replicable fluke). It’s also not a good idea to run an experiment with much larger sample size (yes, this will reduce variance but it won’t get rid of bias in research design, for example when data-gatherers or coders know what they are looking for). The best solution is to step back and rethink the study design with a focus on control of variation.
RW: Anything else you’d like to add?
AG: In many ways, we think traditional statistics, with its context-free focus on distributions and inferences and tests, has been counterproductive to research the human sciences. Here’s the problem: A researcher does a small-N study with noisy measurements, in a setting with high variation. That’s not because the researcher’s a bad guy; there are good reasons for these choices: Small-N is faster, cheaper, and less of a burden on participants; noisy measurements are what happen if you take measurements on people and you’re not really really careful; and high variation is just the way things are for most outcomes of interest. So, the researcher does this study and, through careful analysis (what we might call p-hacking or the garden of forking paths), gets a statistically significant result. The natural attitude is then that noise was not such a problem; after all, the standard error was low enough that the observed result was detected. Thus, retroactively, the researcher decides that the study was just fine. Then, when it does not replicate, lots of scrambling and desperate explanations. But the problem — the original sin, as it were –was the high noise level. It turns out that the attainment of statistical significance cannot and should not be taken as retroactive evidence that a study’s design was efficient for research purposes. And that’s where the “What does not kill my statistical significance makes it stronger” fallacy comes back in.
Like Retraction Watch? Consider making a tax-deductible contribution to support our growth. You can also follow us on Twitter, like us on Facebook, add us to your RSS reader, sign up on our homepage for an email every time there’s a new post, or subscribe to our daily digest. Click here to review our Comments Policy. For a sneak peek at what we’re working on, click here.
Traditional statistics are not counterproductive to research in human sciences, abuse of traditional statistics is. Traditional statistics includes identification of scientifically relevant effect sizes, and calculation of sample sizes necessary to repeatedly and reliably detect differences of scientific relevance, given the amount of noise in the system.
All measured data has noise. It is generally impossible to obtain exactly the same measurement when repeatedly measuring some phenomenon of interest. The mistake far too many scientific researchers make is failing to identify the magnitude of measured effects that really means something, the “effect size of scientific relevance”.
If a drug helped a person live for one extra second, few of us would deem such an effect to be of much scientific relevance. If an engineering manoeuvre caused a car to travel one extra inch per gallon of gas, few of us would find that to be of much relevance. So in any assessment of a scientifically interesting situation, an early exercise must be to figure out what magnitude of effect will mean something useful or relevant.
Such exercises are often not trivial, but they are not impossible, and they are important and unfortunately all too often overlooked.
Once an understanding of what magnitude of effect means something scientifically, or medically, or biologically, depending on the area of research, that effect size of relevance must be compared to the amount of noise present in measurements available. This is commonly referred to as the signal to noise ratio.
If a signal of importance is small compared to the noise present in individual measurements, then a lot of measurements must be taken in order to consistently and reliably detect the effect. If the signal of importance is large compared to the noise present in individual measurements, then a modest number of measurements will suffice to consistently and reliably detect the effect. This phenomenon occurs because the mean of several observations has smaller variability than the individual measurements, provided the distribution of error amounts for the phenomenon under investigation is not too pathological (the error distribution doesn’t have “fat tails” yielding large errors frequently). Under such scenarios, calculating means of several data points gives better estimates of effect sizes because the means are less noisy than any individual observation, and the mean will get closer to the central location of the average individual data point. These are the two central tenets of statistics encapsulated in the ‘Law of Large Numbers’ (the sample mean converges to the true mean for large samples) and the ‘Central Limit Theorem’ (the distribution of the sample mean becomes close to a ‘bell curve’ for large samples).
Now in all but pathological situations, if you collect a large amount of data, then any statistical test will yield a very small p-value. A small p-value in and of itself is not meaningful without comparing the measured effect size to the effect size of scientific relevance. If the measured effect size is considerably smaller than an effect size of scientific relevance, then it doesn’t matter how small the p-value is, the measured effect isn’t of any scientific relevance.
Understanding the relative relation of the effect size of scientific relevance to the amount of noise in the system allows the researcher to calculate how many observations will typically be needed to reliably detect the effect in the system – this exercise is commonly known as a power calculation.
Any scientific study that does not discuss a-priori power calculations or related exercises to determine the amount of data needed to reliably detect the effect size of relevance is an exploratory study and should be so labeled. Merely finding a small p-value does not indicate that something of scientific relevance has been uncovered. Statistical significance should not be confused with scientific relevance. This is the problem underlying so many studies that fail to replicate. They fail to replicate because they were exploratory exercises only, without careful assessment of what effect size means something useful or important, and given the noise in the system, how much data should be collected to reliably detect such differences.
This issue is often skirted because the answer often is that the researcher needs to collect more data than can be afforded with the money and resources at hand. There’s a reason that clinical trials often involve hundreds or thousands of cases, and that decent opinion polls involve thousands of people. That’s the amount of data required to reliably and repeatedly detect an effect of scientifically relevant size.
Small N is faster and cheaper, but generally not sufficient to reliably detect an effect of any scientifically relevant size. Researchers reading a paper with small sample size and no discussion of power considerations should label such studies as exploratory only, and take the findings with a large grain of salt. Publishers and reviewers should insist that such studies be labeled as exploratory exercises.
Researchers who honestly undertake the important exercises of identifying an effect size of scientific relevance, and calculating the sample size required to reliably detect such an effect, and find that the sample size is too large given their available resources should combine resources with other researchers so that they do end up with useful results. We are wasting far too much money and other resources when we allow so many groups of researchers to publish so many small meaningless findings. The waste is readily apparent in the several current studies regarding the replication crisis.
Whatever the observed result, especially any associated with a small p-value, if there is no discussion of what a scientifically relevant effect size is, then labeling the observed effect as somehow meaningful is fallacious, and does not constitute “traditional statistics”.
It’s not small p-values that are the problem, it is this repeated phenomenon of researchers publishing a result with a small p-value with no attendant discussion of whether the result is one of any scientific relevance and whether the appropriate amount of data was collected. This is the phenomenon behind the current replication crisis.
I spent 20 years teaching research methods, saying exactly this. I was a lone voice in the wilderness. Old fashioned concepts of reliability, validity and testing statistical assumptions (including a priori power analysis with some nifty and free online calculators) hinder the race to publication.
Thank you Kathy for your years of effort.
I do believe they will pay off.
I understand feeling as a lone wolf, in the midst of the current fad wherein those who do not really understand statistical discipline find it easy and cool to jump on the p-value bashing bandwagon. In this era of “alternative facts” a vigorous defense of statistical discipline is important.
Collaboration rather than competition is what we need more of in the sciences. When collaborative groups bring fully formed and repeatedly tested scientific findings to print, rather than competing groups racing to publish nonsense first, we will have fewer journals and fewer annual publications, but a much higher percentage of study findings will be well founded. John Ioannidis paper “Why most published research findings are false” will then become an ancient relic.
Two comments: (1) p-values such as the typical 0.05 are significant only in the way that this may have been the 1 out of 20 that achieved a significant result. (2) Therefore, it would be helpful if the to-be-tested hypotheses needed to be specified and documented before the experiment.
My vote is for Steven McKinney. The title of the article is misplaced.
Stephen writes, “Traditional statistics are not counterproductive to research in human sciences, abuse of traditional statistics is.”
To me, “traditional statistics” is what people do. The tradition is not just theorems in a textbook, it’s statistical practice. And a big part of traditional statistics is using hypothesis tests to reject a straw-man null hypothesis and then taking this as evidence in favor of a preferred alternative. This is a particular concern when noise is high, effects are variable, and theories are weak.
Ah, the “straw-man” meme.
In this era of false news I must frequently and vigorously defend effective statistical methodologies that allow us to uncover scientific truths, rather than just publish stuff that somehow feels right. Now more than ever people need to understand which methodologies can help us understand scientific truths.
I appeal to you as a professor of statistics to stop contributing to the current pop-culture bashing of effective statistical methodology. I know it gets lots of hits on your blog site, but we need more than that right now.
I also appeal to you, and Frank Harrell, and David Colquhoun, and others whose articles bashing p-values have recently been linked on Retraction Watch posts and Weekend Reads to stop bashing p-values, then state that Bayesian methods will somehow save us, without presenting any statistically or mathematically rigorous demonstration of the superiority of Bayesian methods. As I have stated in other posts here and elsewhere, most frequentist and Bayesian approaches will converge, yielding the same answer, as sample sizes grow large. In the rare cases where they do not, reasoned comparisons of the approaches has yielded valuable insights into some tricky aspects of assessing evidence in complex situations.
We are frequently faced with a situation of comparing two systems, attempting to assess similarities (often expressed via a null hypothesis positing no difference in some aspect of distributional characteristics) and differences (often expressed via an alternative hypothesis positing some difference). Labeling such components with terms such as “straw-man” suggests to those not well versed in statistical methodology that there is something inappropriate going on. (Dictionary definition of straw man : An insubstantial concept, idea, endeavor or argument, particularly one deliberately set up to be weakly supported, so that it can be easily knocked down; especially to impugn the strength of any related thing or idea.)
What has gone off the rails here is understanding of proper steps in assessing the concordance of evidence from data with underlying statistical frameworks. A single statistical test showing a p-value less than 0.05 does not constitute solid evidence to support a scientific hypothesis. Fisher, the father of modern statistical methods, stated that “A scientific fact should be regarded as experimentally established only if a properly designed experiment rarely fails to give this level of significance.” A single experimental result is not a rare thing. This is why reproducibility concepts are receiving so much focus now.
We have strayed from Fisher’s good guidance, journal editors and reviewers do not insist on multiple demonstrations from properly designed experiments, and this needs to be redressed. Setting up appropriate null and alternative hypotheses, assessing effect sizes of scientific relevance, determining sample sizes to properly assess the concordance of the effect size of relevance with the hypotheses given the noise of the system, then repeatedly demonstrating, all constitute a sound basis for assessing scientific phenomena. I’ve outlined them in some detail above. Chapter 3 of D.R. Cox’s “Principles of Statistical Inference” nicely lists and describes those steps, including reference to the underlying philosophy of severe testing of statistical hypotheses developed over the last 3 decades by Deborah Mayo, that clearly underpin those steps.
Labeling a useful component in the toolkit necessary to redress such issues as a “straw-man” is not helping.
“To me, “traditional statistics” is what people do.” Making up your own definition.
From personal experience with chronic disease in my family, I couldn’t agree more that doctors have very little confidence in their treatments, and many treatments are at best useless, but often counterproductive, mainly because there are many causes for similar conditions and there needs to be several classes of treatment based on the individual. However, this complexity is almost impossible to resolve with traditional statistics.
Andrew, is anyone doing research into creating new forms of statistical analysis that require every measurement to use a 0 to 1 value, so that subtle yet consistent correlations can be revealed? Even better, are there new techniques so that individuals can draw better inferences about what works for *them*?
Andrew, I was a bit surprised, as a researcher in the medical space, to see that anyone even needs to talk about these issues. Evidently the psychology field has an endemic problem with poor research practice. Noise is a fact of life in research, and the critical issue is how one approaches it: data mining is in principle fine, but should be declared as such as a preemptor to conducting a “proper” study. If psychology is conducting non-hypothesis driven data mining as a major mode of generating knowledge, then – yes – time to clean up your house!